Vol: 4 • Issue: 6 • June 2014 [contribute] [archives]
Power users and diversity in WikiProjects; the “network of cultures” in multilingual Wikipedia biographies
With contributions by: Taha Yasseri, Maximilian Klein, Piotr Konieczny, Kim Osman, and Tilman Bayer
Contents
- 1 New book: Global Wikipedia
- 2 “Interactions of cultures and top people of Wikipedia from ranking of 24 language editions”
- 3 “Recommending reference materials in context to facilitate editing Wikipedia”
- 4 “What do Chinese-language microblog users do with Baidu Baike and Chinese Wikipedia?”
- 5 Content or people? Achieving critical mass to promote growth in WikiProjects
- 6 “Prediction of Foreign Box Office Revenues Based on Wikipedia Page Activity”
- 7 Briefly
- 8 Other recent publications
- 9 References
New book: Global Wikipedia
An edited volume[1] by Pnina Fichman and Noriko Hara from Indiana University, Bloomington was released on May 23, 2014, subtitled “International and Cross-cultural Issues in Online Collaboration”. The book description states that “dozens of books about Wikipedia are available, but they all focus on the English Wikipedia and assume an Anglo-Saxon perspective, while disregarding cultural and language variability or multi-cultural collaborative efforts”. The description claims that this is “the first book to address this gap by focusing attention on the global, multilingual, and multicultural aspects of Wikipedia.” The book contains nine chapters authored by 16 Wikipedia researchers (including a chapter authored by the volume editors). Among the topics covered are international and cross-cultural conflict and collaboration, case studies in the Chinese, Finnish, French, and Greek Wikipedias, and Wikipedia gender gaps in different language sites.
“Interactions of cultures and top people of Wikipedia from ranking of 24 language editions”
Review by Maximilianklein (talk)
This research by Eom et al.[2] is an exploratory data analysis of figures (roughly, “people”) from a mining of date and place of birth and gender in biography articles. Presenting novel ideas based on the infamous Google PageRank algorithm, this paper is a sort of computational history. The methods used are standard – if not a bit dated – compared with more contemporary research using Wikidata.
This is a shame because newer techniques would have allowed the claims of a quantified cultural influence factor to rest on firmer grounds.
Their method is for each of their 24 Wikipedia languages (approximately the top 24 largest ones) to construct the network where nodes are biography articles, and links are intrawiki-links. Then they rank each node by both PageRank and 2DRank. PageRank says your importance is a recursive function of your incoming links, weighted by the page rank of each incoming linker; CheiRank is the same as PageRank, but using outgoing links instead. 2DRank is a mixture of PageRank and CheiRank. Some of the authors have coauthored earlier papers that similarly examined PageRank and CheiRank for biographical and other Wikipedia articles (see our previous coverage: “How Wikipedia’s Google matrix differs for politicians and artists” and “Multilingual ranking analysis: Napoleon and Michael Jackson as Wikipedia’s ‘global heroes’“).
However, the input to these algorithms is the weak part. The base set consists of all of the articles that are in a subcategory of Biographies of Living People, Births by Year, or Deaths by Year. Obtaining 1.1 million biography articles, they acknowledge that this isn’t a full set because it is based off English Wikipedia, but then make an anecdotal claim that it’s only 2% off. However, with the latest Wikidata information we know of at least 2.08 million “people” with Wikipedia articles[3].
The rest of their method consists of finding the top 100 articles in each of the 24 languages using both PageRank and 2DRank. Then they get birth place, birthdate and gender from DBpedia if available, and if not they look up this information manually. They pigeonhole each article into one of the 24 target cultures based on birth place, and use a “World” category if none applies. Simplifying assumptions are also made during these processes: modern borders are used, and each country is assumed to speak only a single language. So Kant is Russian and all Belgians speak Dutch in this research.
There is an exploratory analysis of these top 100 by geography, time, and gender. The results confirm a long-told story: the biographies that the English Wikipedia knows about are heavily skewed towards being Western/European, modern, and male. They make points of showing local favour, e.g. Hindi has many in their top 100 who are born in India. With regard to history, the authors note that the Arabic Wikipedia is more interested in history than what world growth would suppose. Another measure is defined to look at the localness factor by decade – that is, what percentage of top figures in this decade were born in this language-place? Of course it’s Greeks early on, and the US dominating later.
On gender, their results indicate 5.1% or 10.1% by PageRank and 2DRank, respectively, are female of the top 100s, averaged. The authors make mention that maleness does decrease over time as well. This reported figure is more severe than the overlap with any single language, so the authors show some “wisdom of the crowds” effect.
The final analysis tries to quantify cultural influence. A “network of cultures” is made, where nodes are each of the 24 languages-cum-cultures, and the directed, weighted edges are the number of foreigners in their top 100. For instance, in the English Wikipedia’s top 100, five people were born in France; so England connects to France with a weight of 5. With this “network of cultures” in hand, they apply the PageRank and 2DRank algorithms to rank each culture. This is a novel approach to making statistical what we all often guess at. Even despite the fact that Jesus is considered Arabic through their simplifications, PageRank turns up English and German as top and runner-up, respectively. Using 2DRank, Greek, French and Russian get more due.
In summary, although this cultural research suffers from biased data, some clever ideas are implemented – particularly the “network of cultures”. The implication is that statistical history somewhat corroborates the opinions of manually conducted history.
“Recommending reference materials in context to facilitate editing Wikipedia”
This article[4] describes IntelWiki, a set of MediaWiki tools designed to facilitate new editor’s engagement by making research easier. The tool “automatically generates resource recommendations, ranks the references based on the occurrence of salient keywords, and allows users to interact with the recommended references within the Wikipedia editor.” The researchers find that volunteers using this tool were more productive, contributing more high-quality text. The studied group was composed of 16 editors with no Wikipedia editing experience, who completed two editing tasks in a sandbox wiki, one using a mockup Wikipedia editing interface and Google search engine, and using the IntelWiki interface and reference search engine. The author’s reference suggestion tool seems valuable, unfortunately this reviewer was unable to locate any proof that the developer engaged the Wikipedia community, or made his code or the tool publicly available for further testing. The research and the thesis does not discuss the differences between their MediaWiki clone and Wikipedia in any significant details. Based on the limited description, the study’s overall conclusions may not be reliable, since the mockup Wikipedia interface used for the comparison seems to be a default MediaWiki clone, lacking many Wikipedia-specific tools; therefore the theme of comparing IntelWiki to Wikipedia is a bit misleading.
While the study is interesting, it is disappointing that the main purpose appears to be completing a thesis,[5] with little thought to actually improving Wikipedia (by developing public tools and/or releasing open code). (See also: related webpage, YouTube video)
“What do Chinese-language microblog users do with Baidu Baike and Chinese Wikipedia?”
This paper [6] (accepted for presentation at OpenSym 2014, and subtitled “A case study of information engagement”) explores the use of the Chinese Wikipedia and Baidu Baike encyclopedia by Chinese microblog (Twitter, Sina Weibo) users through qualitative and quantitative analyses of Chinese microblog postings. Both encyclopedias are often cited by microblog users, and are very popular in China to the extent that the words “wiki” and “baidu” have become verbs meaning to look up content on the respective websites, analogous to “to google” in English.
One of the study’s major focuses is the impact of Internet censorship in China; particularly since Wikipedia is not censored – but access to it, and its discussion in most Chinese websites may be. Baidu Baike is both censored and more likely to host copyright violating content. Despite Baidu Baike’s copyright violating content, many users still prefer the uncensored and more reliable Chinese Wikipedia, though they can become frustrated by not being able to access it due to censorship. Whether some Wikipedia content is censored or not is seen by some as a measure of the topic’s political sensitivity. The author suggests that a distinguishing characteristic can be observed between groups that prefer one encyclopedia over the other, but does not discuss this in detail, suggesting a very interesting research avenue.
Content or people? Achieving critical mass to promote growth in WikiProjects
Review by Kimaus
In a recent paper[7], Jacob Solomon and Rick Wash investigate the question of sustainability in online communities by analysing trends in the growth of WikiProjects. Solomon and Wash track revisions and membership in over one thousand WikiProjects over a period of five years to examine how the concept of a critical mass can influence a community’s development. The key question being, as the title of the paper states: “Critical mass of what?” Is it achieving a certain number of contributions or a certain number of members that will ensure the future sustainability of an online group?
Using critical mass theory, which describes groups as having an accelerating, linear or decelerating production function, the authors modelled a growth curve for each community. They found that the majority of WikiProjects had an accelerating growth regarding the number of revisions, however a decelerating growth in accruing members which suggests that existing editors are increasing individual contributions to the projects. In further examining this trend Solomon and Wash focus on the early years of projects’ existence to determine whether amassing content or editors in this formative period influences future production functions.
Their modelling shows that a greater number and diversity of editors within a project positively affects the number of revisions accumulated after five years (where diversity is calculated through membership in other WikiProjects). Interestingly, the modelling showed contributions by infrequent participants helped a project grow, but this can be offset by “overparticipation from a project’s power users.” They attribute this to members’ feeling that they can make a difference to projects that have diverse and sparse contributions. They do note, however, that increased contributions from power users may simply be an attempt to keep a project afloat, and that this effort is ultimately futile in certain cases. In sum, the authors find that it is a critical mass of people (who hold a variety of skills and knowledge) contributing small amounts in the early stages that positively affects a project’s growth and future sustainability.
“Prediction of Foreign Box Office Revenues Based on Wikipedia Page Activity”
In a paper[8] presented at the ChASM Workshop of WebSci’14, Bloomington, Indiana, this month, de Silva and Compton, have generalised a method, previously introduced by Mestyán, Yasseri, and Kertész (see the newsletter review) to predict the box office revenues of movies based on the Wikipedia edits and page-view counts. Of these two metrics, the new paper considers only the page-view statistics of articles about the movies, but extends the sample of movies to include non-American movies as well. Samples of movies in the US, Japan, Australia, the UK, and Germany are studied. The authors concluded: “although the method proposed by Mestyán et al. predicts films’ opening weekend box office revenues in the United States and Australia with reasonable accuracy, its performance drops significantly when applied to various foreign markets. … we used the model to predict the opening weekend box office revenues generated by films in British, Japanese, and German theatres, [and] found its accuracy to be far from satisfactory.”
Briefly
“Building academic literacy and research skills by contributing to Wikipedia”
A survey[9] of research skills of a group of students at an Australian institution showed that purposeful engaging with Wikipedia, including contributing to it, improved their academic skillset.
“Google and Bing reintroduce national boundaries more so than Wikipedia does”
In a blog post titled “How does Wikipedia cover the world differently than Google (or Bing)?”,[10] researcher Han-Teng Liao examines this question by looking at the case of Belgium, which has several language areas. While the two search engines offer a national portal page (google.be / be.bing.com) in different language options, “Wikipedia organizes its users and information less along the lines of national differences and more along the lines of language differences. According to various traffic reports provided by the Wikimedia foundation, users from Belgium contribute to viewing and editing activities mostly in its Dutch, French and English versions.”
Other recent publications
A list of other recent publications that could not be covered in time for this issue – contributions are always welcome for reviewing or summarizing newly published research.
- “Inferring Semantic Facets of a Music Folksonomy with Wikipedia”[11]
- “Towards linking libraries and Wikipedia: automatic subject indexing of library records with Wikipedia concepts”[12]
- Pandemic page views in online news media and Wikipedia: From the English abstract of this German-language paper[13]: “… a time-series analysis is done comparing the amount of the coverage of eleven online media on the EHEC pandemic in summer 2011 and the amount of page requests for articles in the online encyclopedia Wikipedia relevant to EHEC. Overall, analyses show strong correlations but also temporary discrepancies, appearing because page requests do not only depict the public agenda but also existing uncertainty about an issue.”
- “What Makes a Good Team of Wikipedia Editors? A Preliminary Statistical Analysis”[14]. From the abstract: “The paper concerns studying the quality of teams of Wikipedia authors with statistical approach. […] The analysis confirmed that the key issue significantly influencing article’s quality are discussions between teem [sic] members. The second part of the paper successfully uses machine learning models to predict good articles based on features of the teams that created them.”
- “A computational linguistic approach towards understanding Wikipedia’s article for deletion (AfD) discussions”[15]. From the abstract: “In this thesis we aim to solve two main problems: 1) how to help new users effectively participate in the [deletion] discussion; and 2) how to make it efficient for administrators to make decision based on the discussion. To solve the first problem, we obtain a knowledge repository for new users by recognizing imperatives. We propose a method to detect imperatives based on syntactic analysis of the texts. And the result shows a good precision and reasonable recall. To solve the second problem, we propose a decision making support system that provides administrators with an reorganized overview of a discussion.”
References
- ↑ Fichman, Pnina (2014). Global Wikipedia : international and cross-cultural issues in online collaboration. Lanham: Rowman & Littlefield. ISBN 9780810891012.
- ↑ Eom, Young-Ho; Pablo Aragón, David Laniado, Andreas Kaltenbrunner, Sebastiano Vigna, Dima L. Shepelyansky (2014-05-28). “Interactions of cultures and top people of Wikipedia from ranking of 24 language editions“. arXiv:1405.7183 [physics].
- ↑ Sex Ratios in Wikidata.
- ↑ Mohammad Noor Nawaz and Andrea Bunt (2014) IntelWiki: recommending resources to help users contribute to Wikipedia. In Proceedings of the 22nd International Conference on User Modeling, Adaptation, and Personalization (UMAP 2014), 12 pp., forthcoming. PDF
- ↑ [1]
- ↑ Liao, Han-Teng (2014-06-17). What do Chinese-language microblog users do with Baidu Baike and Chinese Wikipedia?.
- ↑ Solomon, Jacob; Rick Wash (2014-05-16). “Critical mass of what? Exploring community growth in WikiProjects”. Eighth International AAAI Conference on Weblogs and Social Media. Eighth International AAAI Conference on Weblogs and Social Media. http://www.aaai.org/ocs/index.php/ICWSM/ICWSM14/paper/view/8104.
- ↑ de Silva, Brian; Ryan Compton (2014-05-22). “Prediction of foreign box office revenues based on wikipedia page activity“. arXiv:1405.5924 [physics].
- ↑ Miller, Julia (2014-06-13). “Building academic literacy and research skills by contributing to Wikipedia: A case study at an Australian university“. Journal of Academic Language and Learning 8 (2): A72-A86. ISSN 1835-5196.
- ↑ Liao, Han-Teng (2014-05-13). How does Wikipedia cover the world differently than Google (or Bing)?.
- ↑ Sordo, Mohamed; Fabien Gouyon, Luís Sarmento, Òscar Celma, Xavier Serra (2013). “Inferring semantic facets of a music folksonomy with Wikipedia“. Journal of New Music Research 42 (4): 346-363. doi:10.1080/09298215.2013.848904. ISSN 0929-8215. Retrieved on 2014-06-28.
- ↑ Joorabchi, Arash; Abdulhussain E. Mahdi (2014-04-01). “Towards linking libraries and Wikipedia: automatic subject indexing of library records with Wikipedia concepts“. Journal of Information Science 40 (2): 211-221. doi:10.1177/0165551513514932. ISSN 0165-5515. Retrieved on 2014-06-28.
- ↑ Holbach, Thomas; Dr Marcus Maurer. “Wissenswerte Nachrichten“. Publizistik: 1-17. doi:10.1007/s11616-013-0191-z. ISSN 0033-4006.
- ↑ Bukowski, Leszek; Michał‚ Jankowski-Lorek, Szymon Jaroszewicz, Marcin Sydow (2014-01-01). “What Makes a Good Team of Wikipedia Editors? A Preliminary Statistical Analysis”. In Akiyo Nadamoto, Adam Jatowt, Adam Wierzbicki, Jochen L. Leidner (eds.). Social Informatics. Lecture Notes in Computer Science. Springer Berlin Heidelberg. pp. 14-28. ISBN 978-3-642-55284-7.
- ↑ Mao, Wanting. A computational linguistic approach towards understanding Wikipedia’s article for deletion (AfD) discussions. Master’s thesis The University of Western Ontario, 2014. PDF
Wikimedia Research Newsletter
Vol: 4 • Issue: 6 • June 2014
This newletter is brought to you by the Wikimedia Research Committee and The Signpost
Subscribe: Email
• [archives] [signpost edition] [contribute] [research index]
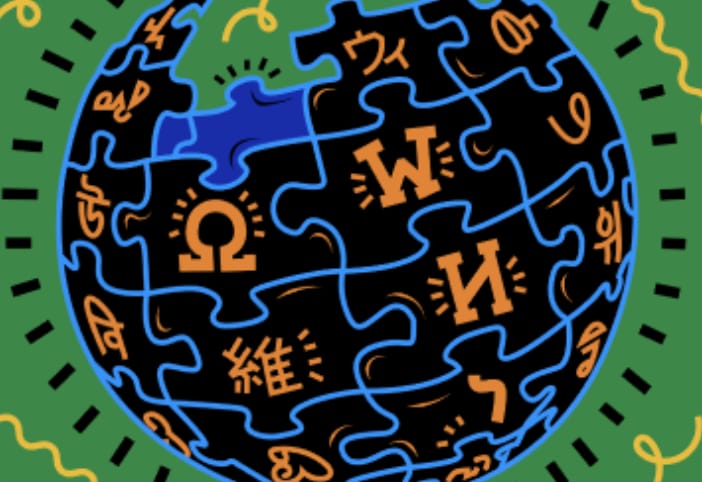
Can you help us translate this article?
In order for this article to reach as many people as possible we would like your help. Can you translate this article to get the message out?
Start translation