Taken together, these facts point to a problem: Edit reviewers need powerful tools to handle the avalanche of wiki edits, but power tools can do damage to good-faith newcomers. Is there a way to help edit reviewers work more efficiently while also protecting newbies?
That’s precisely the question that’s been driving the Foundation’s Collaboration team over the past months. We think the answer is yes, and we took a big step toward making good on that conviction with the recent release of the new filters for edit review beta. The beta adds a suite of new tools to the recent changes page and introduces an improved filtering interface that’s more user friendly yet also more powerful. Of special note are two sets of filters that let patrollers on several wikis leverage advanced machine learning technology with new ease.
Work smarter
The AI-powered contribution quality filters make predictions about which edits will be good and which will have problems.” Here, a reviewer filters broadly for edits that “May have problems.” At the same time, she uses colored highlighting to emphasize the worst or most obviously bad edits (in yellow and orange). The user is in the process of adding a red highlight to accentuate “Likely bad faith.”
———
Recent changes is essentially a search page. By helping patrollers more effectively zero in on the edits they’re looking for, the New Filters beta can save them time and effort. It does this in a number of ways.
Quest for quality: Over the years, reviewers have developed numerous techniques for picking out edits most in need of examination. Contribution quality prediction filters take this to a new level of sophistication. Powered by the machine learning service ORES, they offer probabilistic predictions about which edits are likely to be good and which may, as the system diplomatically puts it, “have problems.” (“Problems” here can be anything from outright vandalism to simple formatting errors.) Armed with these predictions, edit reviewers can focus their efforts where they’re most needed.
The quality prediction filters make use of scores from ORES’ damaging test and are available only on wikis that support this function. Subscribers to the ORES beta feature have seen damaging scores before, but the new beta deploys the scores very differently. Reviewers now rank edits using a series of up to four filters that offer choices ranging from “Very likely good” to “Very likely have problems” (see illustration).[1]
Figuring out how to present sophisticated artificial intelligence functions in ways that users find clear and helpful was a big focus for the project team during design and user testing. Going forward, we plan to standardize the new approach wherever possible across tools and pages that use ORES.
Use Highlighting to pick out the edit qualities that interest you most. Here, the user filters for edits that are “Very likely good faith.” With colors, she spotlights new pages (yellow) and edits by new users (green and blue). Note the yellow-green row at bottom; the blended color and the two colored dots in the left margin signify that the edit is both a “Page creation” and by a “Newcomer.”
———
Hit the highlights: Expert patrollers have an amazing ability to scan a long list of edit results and pick out the vital details. For the rest of us, however, recent changes search results can present as dense and confusing walls of data. This is where the new highlighting function can be helpful.
Highlighting lets reviewers use color to emphasize the edit properties that are most important for their work. In the illustration above, for example, the reviewer is interested in new pages by new users. By adding a new layer of meaning to search results, Highlighting, again, lets reviewers better target their efforts (learn more about highlighting).
About face: The old recent changes interface had grown incrementally over the years, like the layers of an ancient city. It could be hard to understand, and in testing we found that many users just ignored the many functions on offer. Slapping yet another layer of tools on top of the pile would, we judged, only add to the confusion.
The new interface is designed to help users by giving them useful feedback. Here, the system instructs the user about selected filters that are in conflict.
———
Accordingly, designer Pau Giner completely reimagined the interface to make it both friendlier than the existing one and more powerful. Functions are grouped logically and explained more clearly. A special “active filter” area makes it easy for users to see what settings are in effect. And the system is smart about providing helpful messages that clarify how tools interact (see illustration).
Under the hood, the filtering logic has been reorganized and extended, so that users can more precisely specify the edit qualities they want to include or exclude. (Without going into the details, suffice it to say that users now refine their searches in a manner that will feel familiar from popular shopping and other search-based sites. Learn more about the filtering interface.)
Find the good
Recent changes patrolling has, understandably, always focused more on finding problems and bad actors. But a number of new search tools let reviewers seek out positive contributions. The “very likely good” quality filter, for example, is highly accurate at identifying valid edits. Two other new toolsets are aimed particularly at finding new contributors who are acting in good faith.
For all intents: User intent predictions filters add a whole new dimension to edit reviewing. Powered, like the quality filters, by machine learning (and, like the quality filters, available only on certain wikis), they predict whether a given edit was made in good or bad faith.
Hold on, you might be thinking: intent is a state of mind. How can a computer judge a mental, and even moral state? The answer is that the ORES service has to be trained by humans—specifically, by volunteer wiki editors, who judge a very large sample set of real edits drawn from their respective wikis. These scored edits are then fed back into the ORES program, which uses the patterns it detects to generate probabilistic predictions. (This page explains the training process and how you can get it started on your wiki.) The new interface ranks these predictions into four possible grades, ranging from “very likely good faith” to “very likely bad faith.”
The intent filters are new, and it remains to be seen just how patrollers will put them to use. Clearly, a bad-faith prediction will act as an additional red flag for vandalism fighters. We’re hoping that the good-faith predictions will prove useful to people who want to do things like welcome newcomers, to recruit likely candidates for WikiProjects and to generally give aid and advice to those who are trying, however unskillfully, to contribute to the wikis .
Are you experienced: The New Filters beta also adds a set of “Experience level” filters that identify edits by three newly defined classes of contributors.
- Newcomers have fewer than 10 edits and 4 days of activity. (Research suggests that it’s these very new editors who are the most vulnerable to harsh reviews.)
- Learners have more experience than newcomers but less than experienced users (this level corresponds to autoconfirmed status on English Wikipedia).
- Experienced users have more than 30 days of activity and 500 edits (corresponding to English Wikipedia’s extended confirmed status).
More to come
“New filters for edit review” is very much in development; over the next few months, Collaboration Team will stay focused on fixing problems and adding new features. Among the improvements on our task list: Incorporate all existing recent changes tools into the new interface; add a way for users to save settings; add additional filters users are requesting. Then, after another round of user testing, the plan is to bring the new tools and interface to watchlists.
As always, we need your ideas and input to succeed. To try the new filters for edit review, go to your beta preferences page on a Wikimedia wiki and opt in. Then, please let us know what works for you and what could be better. We’re listening!
Footnote
[1] The number of filters available varies from wiki to wiki, to account for variations in how good ORES’ predictions are on different wikis. The better ORES performs on a wiki, the fewer filter levels are needed. When performance is not as high, additional filter levels enable users to balance performance tradeoffs in ways that work best for them. The quality and intent filters page introduces the concepts behind this.
Joe Matazzoni, Product Manager, Collaboration, Editing Product
Wikimedia Foundation
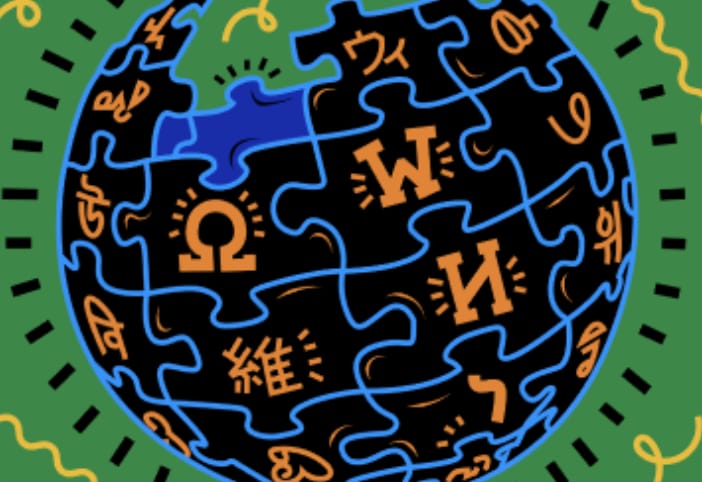
Can you help us translate this article?
In order for this article to reach as many people as possible we would like your help. Can you translate this article to get the message out?
Start translation