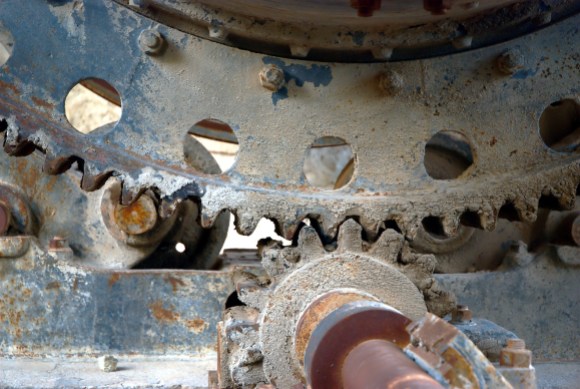
Editor’s note: ORES makes predictions about which edits are vandalism, which new page creations are problematic, and which articles are ready to be nominated for Featured status. (See our past posts about how it works and how to measure content gaps with it)
You can’t prevent bad things from happening. Something will always go wrong. So you do the best that you can to handle bad things gracefully. In a distributed processing environment like ORES’ cluster, the worst thing that could happen is to have a process blocked for forever. So, preparing for bad things means you use timeouts for just about everything. So far, this has been a great strategy and it makes it so that, at worst, only a few requests out of many will fail when something goes wrong. Regretfully, for the downtime event on June 23, we had one of the worst bad things happen, and at the same time we discovered that our timeouts were not capable of stopping deep processes that go rogue in a specific way. In this blog post, I’ll explain what happened.
Recursive backtracking in a regular expression
Many of the models deployed in ORES use regular expressions to extract signal about the quality of an edit. For example, we use them to match “bad words” (curse words, racial slurs, and other words that are commonly used to cause offense) and “informals” (linguistic colloquialisms like “haha” or “lol” or “brb”). One such regular expression that we used to match informal laughing in Spanish language looked like this: /j+[eaiou]+(j+[aeiou]*)*/ It is intended to match strings like “jajajaja”—the Spanish version of “haha”—or “jijiji”.
In this edit of Spanish Wikipedia, an IP editor added a very long string of repeated “JAJAJJAJAJAJAJAJAJ” to the article for “Terrain“. This is exactly what the regular expression was designed to match. But there was a problem. This regular expression was poorly designed in that it caused a catastrophic backtracking pattern. Every time it would match the entire sequence of “JAJAJJAJAJAJAJAJAJ” and then fail when encountered “…JAJAJlentos…” at the end of the sequence, it would re-attempt the entire match dropping just one “JA” from the middle. This problem doesn’t really matter for any short sequences because computers are fast. But for a very long sequence (and this one was 4155 chars long == 230 repetitions of “JAJAJJAJAJAJAJAJAJ”), it would have taken days to finish. The plot below demonstrates how badly things break down at only 14 repetitions.
A plot of the time spent waiting for a regex match for varying repetitions of “JAJAJA…” demonstrates an exponential effect. The graph cuts off at 14 repetitions, but the edit that took ORES down repeated 230 times. 15 seconds is ORES’ default timeout. Image by Aaron Halfaker, CC BY-SA 4.0.
Where were the timeouts?
Things like this happen. When operating in a distributed processing environment, you should always have timeouts on everything so that when one process goes haywire, it doesn’t take everything else down with it. Regretfully, matching a regular expression is not just a special opportunity for pathological backtracking, but also an opportunity for us to learn hard lessons about safe and effective timeouts.
We have timeouts in ORES in a few strategic places. E.g. if a single scoring job takes longer than 15 seconds (extracting informal “JAJAJA” is part of a scoring job), then it is supposed to time out. But for some reason, we weren’t timing out during regular expression matching. I started digging into the library we use to implement execution timeouts, and what I learned was horrifying.
Most timeouts in python are implemented with “threads”. I put “threads” in quotes because threads in python are a convenient abstraction and not true concurrency. Python’s Global Interprer Lock (GIL) is an internal lock that prevents truly concurrent threading. In order to get around this, python uses separate processes to implement concurrency (see the multiprocessing library). I’m not going to get into the details of the GIL or process based concurrency, but suffice it to say, if you use an external C library to execute a regular expression match on a string, any “thread” that is trying to implement a timeout is going to get locked up and totally fail to do what it is supposed to do!
Because our threading-based timeouts were completely disabled by this long regular expression match, our “precaching” system (a listening process that makes sure we score every edit to Wikipedia as it happens) was slowly taking us down. Every time the problematic diff was requested, it would render yet another worker unreachable. Because ORES would then just fail to respond, our precaching system registered a Connection Timeout and would simply retry the request. Eventually capacity would decay as our ~200 workers were locking at 100% CPU one by one — trying to match “JAJAJJAJAJAJAJAJAJ” times 230.
Luckily, there’s an easy solution to this problem in unix signals. By having the operating system help us manage our timeouts, we could stop relying on python threads to behave sanely in order for us to recover from future rogue processes.
So, you fixed it right?
First, I should thank @ssastry for his quick work identifying the pathological backtracking problem and submitting a fix. We also completed an emergency deployment of ORES that implemented the use of Unix signals and we’ve been humming along, scoring all of the things, ever since. It’s times like these that I’d like to advocate for public incident reporting. By publicly acknowledging the problem and discussing the solutions we’ve implemented, we have a better shot at both making sure this doesn’t happen again and informing other so they can maybe avoid our mistakes. We strive to make sure that ORES is a useful and stable utility for Wikipedians.
Aaron Halfaker, Principal Research Scientist
Wikimedia Foundation
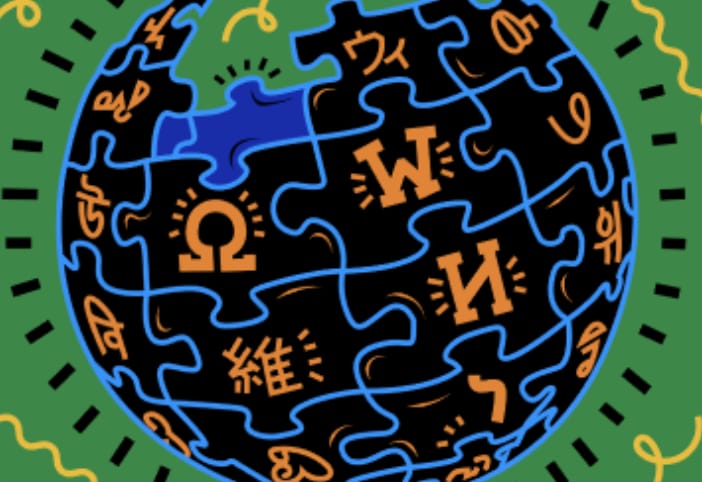
Can you help us translate this article?
In order for this article to reach as many people as possible we would like your help. Can you translate this article to get the message out?
Start translation