Contents
Who wrote this? A new dataset tracks the provenance of English Wikipedia text over 15 years
Much of the existing Wikipedia research is based on the freely licensed datasets published by the Wikimedia Foundation: Content dumps, pageview numbers, Clickstream samples, etc. But some individual researchers are giving back too. An example for this is the TokTrack dataset, described in an accompanying paper[1] as
- “a dataset that contains every instance of all tokens (≈ words) ever written in undeleted, non-redirect English Wikipedia articles until October 2016, in total 13,545,349,787 instances. Each token is annotated with (i) the article revision it was originally created in, and (ii) lists with all the revisions in which the token was ever deleted and (potentially) re-added and re-deleted from its article, enabling a complete and straightforward tracking of its history.”
Tracking authorship and provenance of Wikipedia article text is by no means a new topic (see e.g. Research:Content persistence). However, the paper’s authors assert that their method provides much higher accuracy than earlier efforts such as Wikitrust. One of them, Fabian Flöck, has been studying this problem with other researchers for years (cf. our coverage from 2012 and 2014: “Precise and efficient attribution of authorship of revisioned content”, “Better authorship detection, and measuring inequality“, “New algorithm provides better revert detection“; the present dataset is generated by their “Wikiwho” algorithm, which also underlies a browser extension called “Whocolor”).
What’s more, the papers points out that “this data would be exceedingly hard to create by an average potential user” for the entire English Wikipedia due to the computational effort involved (“around 25 days on a dedicated Ubuntu Server […] with 122 GB RAM and 20 cores”; for comparison, a community-created tool, “WikiBlame“, which is linked from every revision history page on English Wikipedia, can take several minutes to find the provenance of an individual token in a single article).
After describing the dataset and the underlying methodology, the paper also briefly presents some insights that can be derived from it about the history of English Wikipedia. First, it looks at the number of added and surviving tokens over time, observing that
- “the rapid growth in added tokens leveled off around the beginning of 2007, and transformed into a slight decline before recovering towards the middle of 2014. […] the ratio of newly added content that was good or uncontentious enough to survive 48 hours exhibits a (mostly) continuous decrease from 2001 until 2007, coinciding with the change in total added content, then stabilizes and even begins to slightly climb again until recently.”
It highlights “a surprising spike in Oct. 2002 (also in absolute additions)”. Although not mentioned in the paper, this is very likely the effect of bot contributions by en:User:Ram-Man of US geographical content. Figure 2(b) in the paper also seems to indicate that more than half of these October 2002 additions were still live 14 years later.
Analyzing the “persisting” tokens (that had not been removed within 48 hours) by user group, the authors observe:
- “While it seems that the addition of persisting tokens of unregistered editors has become comparably stable since 2006, it has not been keeping up by far with the enormous increase by registered editors, which make up for over 80% of all added surviving content for most months since 2007. In fact, a small group of registered users generates the vast majority of sustained content […. Bots showed an increased presence from mid-2007 until 2013, when, presumably by the migration of inter-language links to Wikidata, the demand for bot-created content dropped.”
The remainder of the paper uses the dataset to study editing controversies. First, the authors define two measures of how controversial an article is, both yielding evolution, Mustafa Kemal Atatürk and Bob Dylan (in that order) as the three most controversial articles as of October 2016 (based on the surviving content at that time only). They also find that “barneys” was the top most conflicted string token. Lastly, they examine the frequency of edits that undo other edits partially or totally, where the token-based data enables a more sophisticated approach than simpler types of revert analysis. They find that
- “in total, 61.51% of all edits included some kind of removal or reinsertion of content (i.e., 38.49% revisions purely added content), and in 14.62% of the revisions editors correct their own edits. 14.84% of all revisions fully undid another revision and 50.65% did so partially.”
However, they caution that since “content added by one revision can over (a long) time be corroded by many small changes […] ‘revert’ cannot per se be equated with antagonism here, as these numbers include the complete spectrum from minor corrections to full-on opinion clashes and vandal fighting.”
Briefly
Conferences and events
See the research events page on Meta-wiki for upcoming conferences and events, including submission deadlines.
Other recent publications
Other recent publications that could not be covered in time for this issue include the items listed below. contributions are always welcome for reviewing or summarizing newly published research.
- “Public artworks and the freedom of panorama controversy: a case of Wikimedia influence”[2] From the abstract: “Freedom of panorama, an exception to copyright law, is the legal right, in some countries, to publish pictures of artworks which are in public space. A controversy emerged at the time of the discussions towards the revision of the 2001 European Copyright Directive […]. The article decrypts the legal framework and political implications of a topic which has been polarising copyright reform lobbyists, and analyses its development within the public debate since the XIXth century.” From the paper: “The analysis of the media and the lobbying campaign reveal that the main actors of the debate are collecting societies, whose revenues would be affected should the exception become compulsory, or larger. The users’ side, the analysis finds, is mainly represented by Wikipedia, an organisation at the forefront of the campaign and therefore also of this paper. While other stakeholders of the online space are affected by this legal prerogative, neither social media platforms, nor the press, chose to join the campaign towards a new or broader exception.”
- “Analysing temporal evolution of interlingual Wikipedia article pairs”[3] From the abstract: “…we present MultiWiki online demo – a novel web-based user interface that provides an overview of the similarities and differences across the article pairs originating from different language editions on a timeline. MultiWiki enables users to observe the changes in the interlingual article similarity over time and to perform a detailed visual comparison of the article snapshots at a particular time point.”
- “A productive clash of perspectives? The interplay between articles’ and authors’ perspectives and their impact on Wikipedia edits in a controversial domain”[4] From the abstract: “We chose a corpus of articles in the German-language version of Wikipedia about alternative medicine as a representative controversial issue. We extracted edits made until March 2013 and categorized them using a supervised machine learning setup as either being pro conventional medicine, pro alternative medicine, or neutral.”
- “Glaring chemical errors persist for years on Wikipedia”[5] From the abstract: “Though Wikipedia does not require the vetting of submitted material, it is undoubtedly a major resource for the 21st century chemist. Unfortunately, many errors in chemical structure are present in Wikipedia chemistry articles. Even when these mistakes are discovered and reported, articles are sometimes subsequently left uncorrected. In order to be a more useful resource, particularly for undergraduate learning, Wikipedia depends on timely fact-checking and editing by the chemical community.”
- “Emotional content in Wikipedia articles on negative man-made and nature-made events”[6] From the abstract: “… we expected that Wikipedia articles on terrorist attacks contain more anger-related and less sadness-related content than articles on earthquakes. We analyzed newly created Wikipedia articles about the two events (Study 1) as well as more current versions of those Wikipedia articles after the events had already happened (Study 2). The results supported our expectations. Surprisingly, Wikipedia articles on those two events contained more emotional content than related Wikipedia talk pages.”
- “Powerful structure: inspecting infrastructures of information organization in Wikimedia Foundation projects”[7] From the abstract: “This dissertation … analyzes the diverse strategies that members of Wikimedia Foundation (WMF) project communities use to organize information. Key findings from this dissertation show that conceptual structures of information organization are encoded into the infrastructure of WMF projects. […]. I use three methods in this dissertation. I conduct a qualitative content analysis of the discussions surrounding the design, implementation and evaluation of the category system; a quantitative analysis using descriptive statistics of patterns of editing among editors who contributed to the code of templates for information boxes; and a close reading of the infrastructure used to create the category system, the infobox templates, and the knowledge base of structured data.”
- “Implementation and evaluation of a framework to calculate impact measures for Wikipedia authors”[8] From the abstract: ” …ranking Wikipedia authors by calculating impact measures based on the edit history can help to identify reputational users or harmful activity such as vandalism …. However, processing millions of edits on one system can take a long time. The author implements an open source framework to calculate such rankings in a distributed way (MapReduce) and evaluates its performance on various sized datasets.”
- “280 birds with one stone: inducing multilingual taxonomies from Wikipedia using character-level classification”[9] From the abstract: ” Given an English taxonomy, our approach leverages the interlanguage links of Wikipedia followed by character-level classifiers to induce high-precision, high-coverage taxonomies in other languages. Through experiments, we demonstrate that our approach significantly outperforms the state-of-the-art, heuristics-heavy approaches for six languages. As a consequence of our work, we release presumably the largest and the most accurate multilingual taxonomic resource spanning over 280 languages.”
- “Estimating the quality of articles in Russian Wikipedia using the logical-linguistic model of fact extraction”[10]From the abstract: “We present the method of estimating the quality of articles in Russian Wikipedia that is based on counting the number of facts in the article. For calculating the number of facts we use our logical-linguistic model of fact extraction. […] We experimentally compare the effect of the density of these types of facts on the quality of articles in Russian Wikipedia. Better articles tend to have a higher density of facts.”
References
- ↑ Flöck, Fabian; Erdogan, Kenan; Acosta, Maribel (2017-05-03). TokTrack: A Complete Token Provenance and Change Tracking Dataset for the English Wikipedia. Eleventh International AAAI Conference on Web and Social Media.
- ↑ Rosnay, Mélanie Dulong de; Langlais, Pierre-Carl (2017-02-16). “Public artworks and the freedom of panorama controversy: a case of Wikimedia influence”. Internet Policy Review. ISSN 2197-6775.
- ↑ Gottschalk, Simon; Demidova, Elena (2016). Analysing temporal evolution of interlingual Wikipedia article pairs. SIGIR ’16. New York, NY, USA: ACM. pp. 1089–1092. ISBN 9781450340694. doi:10.1145/2911451.2911472.
, eprint: Gottschalk, Simon; Demidova, Elena (2016). “Analysing Temporal Evolution of Interlingual Wikipedia Article Pairs”. arXiv:1702.00716 [cs]: 1089–1092. doi:10.1145/2911451.2911472., Online demo
- ↑ Jirschitzka, Jens; Kimmerle, Joachim; Halatchliyski, Iassen; Hancke, Julia; Meurers, Detmar; Cress, Ulrike (2017-06-02). “A productive clash of perspectives? The interplay between articles’ and authors’ perspectives and their impact on Wikipedia edits in a controversial domain”. PLOS ONE 12 (6): –0178985. ISSN 1932-6203. doi:10.1371/journal.pone.0178985.
- ↑ Mandler, Michael D. (2017-01-26). “Glaring chemical errors persist for years on Wikipedia”. Journal of Chemical Education. ISSN 0021-9584. doi:10.1021/acs.jchemed.6b00478. (letter)
- ↑ Greving, Hannah; Oeberst, Aileen; Kimmerle, Joachim; Cress, Ulrike (2017-06-29). “Emotional content in Wikipedia articles on negative man-made and nature-made events”. Journal of Language and Social Psychology: 0261927–17717568. ISSN 0261-927X. doi:10.1177/0261927X17717568.
- ↑ Thornton, Katherine (2017-02-14). “Powerful Structure: Inspecting Infrastructures of Information Organization in Wikimedia Foundation Projects”. (dissertation)
- ↑ Neef, Sebastian (2017-08-26). “Implementation and evaluation of a framework to calculate impact measures for Wikipedia authors”. arXiv:1709.01142 [cs].
- ↑ Gupta, Amit; Lebret, Rémi; Harkous, Hamza; Aberer, Karl (2017-04-25). “280 Birds with one stone: inducing multilingual taxonomies from Wikipedia using character-level classification”. arXiv:1704.07624 [cs].
- ↑ Khairova, Nina; Lewoniewski, Włodzimierz; Węcel, Krzysztof (2017-06-28). Estimating the quality of articles in Russian Wikipedia using the logical-linguistic model of fact extraction. International Conference on Business Information Systems. Lecture Notes in Business Information Processing. Springer, Cham. pp. 28–40. ISBN 9783319593357. doi:10.1007/978-3-319-59336-4_3.
Wikimedia Research Newsletter
Vol: 7 • Issue: 8 • August 2017
This newsletter is brought to you by the Wikimedia Research Committee and The Signpost
Subscribe: Email
• [archives] [signpost edition] [contribute] [research index]
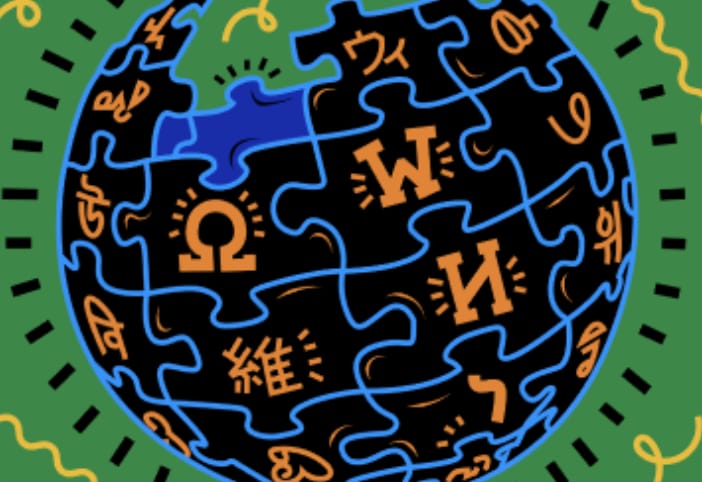
Can you help us translate this article?
In order for this article to reach as many people as possible we would like your help. Can you translate this article to get the message out?
Start translation