Many people worry—some a little, some a lot—about how other people find information on Wikipedia and its sister projects. It’s a driving concern for me and other members of the Search Platform team, and also for many community members, like those who create and curate redirects.
But the curse of knowledge makes it difficult for more sophisticated Wikipedians to put themselves in the shoes of a wiki newbie who is confused by the sea of blue links sprinkled with red links, and who doesn’t yet fully understand notability, naming conventions, talk pages, or search.
What do such newbies need, how do they look for it, and can we help them find it‽
Unearth and extract!
This vicarious fear of missing out leads many people to an awesome idea; it’s an idea that I myself had shortly after I joined the Foundation; it’s an idea others on my team had had a while before I joined them; it’s an idea that many people have proposed and re-proposed and debated and argued about and not quite come to fisticuffs over:
Let’s mine the most frequent queries that get no results, figure out what users are looking for, and arrange to provide it to them—possibly in the form of new articles or redirects from the failed query terms to appropriate existing articles.
It seems like it should be an easy win: Wikipedia gets a steady stream of useful new information, fewer searches get no results at all, and fewer newbies fail to find what they are looking for. But, as is so common, things are more complicated than they first appear.
Privacy, publicity, and prankery
The most important issue is privacy. People sometimes accidentally reveal their private information in their searches. A name, email address, social media account, phone number, physical address, IP address, national ID number, credit card number, love letter, secret recipe, etc., etc.—all can be inadvertently copied from somewhere and pasted into a search box. And, given the million of users of hundreds of Wikipedias and their sister projects, it not only can happen, it does happen—fairly regularly. So, a big raw dump of search data is out of the question.
Several automated methods of preventing the exposure of personal information have been suggested, and many may even be 99.9% effective. The problem is that a 0.1% failure rate multiplied by millions of cases is still thousands of potential privacy problems, when even one is too many.
It is possible to use patterns to identify likely personal information like email addresses, physical addresses, and phone numbers, but errors, unexpected formats, and unknown types of data mean there is always something that can slip through.
Another proposed approach is to require a minimum number of distinct searchers (i.e., distinct IP addresses). After all, if a hundred people have searched for the same thing, it must actually be a thing, right? But a well-placed link on social media (say, in a blog post) can easily generate hundreds or thousands of queries, because some people will click on almost anything.
Since Wikipedia is a such a high-profile website, it’s easy to imagine people trying to game such a system, whether for the fleeting fame or just “for the lulz”. Aspiring celebrities could easily mobilize a relatively small number of fans to propel their brand to the top of such a list. And you never know what will strike the internet’s fickle fancy—which is how we got Boaty McBoatface. Roving bands of internet trolls have famously done much worse in online polls for Mountain Dew in 2012, the Time 100 list in 2009, and countless others.[1]
Diamond in the rough or needle in a haystack?
Thus, while the expected return on mining the most frequent failed queries is often high, the potential risk is also large. Last summer I decided to do a little data spelunking to determine whether not pursuing such prospecting was foolishly abandoning a mountain of hidden gems or wisely avoiding sifting through so much digital dung.
I collected a month’s worth of failed queries from English Wikipedia in May 2016 and tried to filter probable bots and other likely outliers. The result was a corpus of about 8.6 million queries, with 7.4 million unique queries. I carefully reviewed the top 100 most frequent queries, and skimmed the top 1,000.
What I found, mostly, was porn.
The most common query is the name of a porn site. It always shows up in my data when I look at poorly performing queries. In this case, I found five variants of it in the top 100, and they account for about 29% of the raw query count for the top 100.
Other common categories for these searches are other websites, internet memes, TV shows and movies, internet personalities, porn stars, people mentioned in recent news, historical figures, politicians, etc.
I don’t have any opinions on the notability of any particular person or website, but while some of these are clearly popular (based on searches), they have been deemed not notable by the English Wikipedia community. The most frequent porn site, the two most frequent internet personalities, a couple of other sites, and a couple of the internet memes show up in the deletion logs as having been created—some of them multiple times—and deleted. Two articles (with different capitalization) for the most frequent porn site were deleted in 2007, 2009, 2011, 2015, and 2016.
There were also a few typos, but almost all of them were corrected by the completion suggester (suggestions made while you type) and the “did you mean” spelling correction (suggestions made after you submit your query).
Small value, diminishing returns
Over an entire month, only 281 distinct queries passed the proposed 100-unique-IPs threshold, and the 1000th most-frequent query was only searched for 48 times.
The top 100 most frequent queries only accounted for 0.62% of the 8.7M queries, and the top 1,000 only accounted for 1.45% of all queries. The top 25,000 most frequent queries didn’t quite account for 5% of all the queries, and 25,000th had a frequency of 7. The long tail is very long.
In the top 1000, there were at least 10 obvious addresses, a phone number, an ISBN number, an obfuscated email address, a Twitter account, and two instances of a person + city—so aggregating over longer time periods than a month does increase the likelihood of personal data making it past an IP-count filter, validating our privacy concerns.
I gathered the same data for the following month, June 2016, and found it to be very similar. The top 10 most frequent queries were the same as in May, and 71 of the top 100 were the same, indicating that there isn’t a lot of new information at month-over-month timescales.
Some of the same street addresses from May showed up in the June data, indicating to me that the query is likely coming from a link on the internet, or a better class of bot.
Interestingly, the Brexit vote took place in June 2016, and I found 8 misspellings of Brexit in the top 1000 for June—though none in the top 100. Most were corrected by either the completion suggester or the “did you mean” spelling correction.
You can find even more detail about all this in the write up I did last summer.
What went wrong right?
Great minds think alike—so everyone who thought this sounded like a promising idea should give themselves a pat on the back, because lots of other smart people thought so, too.
So what went wrong? Nothing! What went right? My intuition is that, at least on English Wikipedia, the WikiGnomes are so far ahead of the curve that they render this mining exercise moot. There are already so many carefully created and curated articles and redirects that the vast majority of queries that otherwise would have failed, don’t. (CirrusSearch, which the Search Platform hobgoblins work on tirelessly, probably helps a bit, too.)
For other large wikis, particularly Wikipedias, I would expect similar results. Smaller wikis may have a better failed-query gem-to-dung ratio than English Wikipedia, but privacy concerns are still paramount, and the long tail is probably still very, very long.
Trey Jones, Senior Software Engineer, Search Platform
Wikimedia Foundation
Footnotes
- Don’t feed the trolls! That is, no links will be provided. The information is easily found, but also simultaneously tasteless and distasteful, inappropriate for polite conversation, and generally not safe for work.
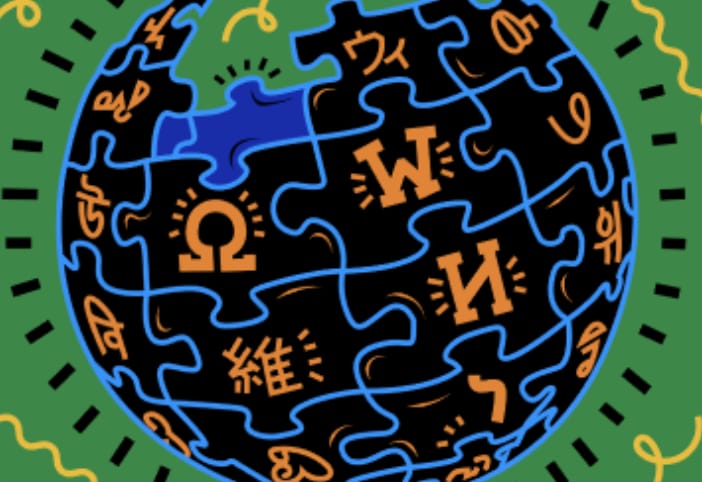
Can you help us translate this article?
In order for this article to reach as many people as possible we would like your help. Can you translate this article to get the message out?
Start translation