Wikidata is a multilingual project by design. The project allows contributors to add structured knowledge in every human language, and acts as a central repository of structured data for Wikipedia and its sister projects. As powerful tools to share knowledge without language barriers, images are very important within Wikidata.
Images can also help illustrate the content of an item in a language-agnostic way to external data consumers. However, a large proportion of Wikidata items lack images: for example, as of today, more than 3.6 million Wikidata items are about humans but only 17 percent of them have an image. More generally, only 2.5 million of 45 million Wikidata items have an image attached.
We recently started a research project to help people find relevant images to add to Wikidata items. The project uses algorithmic image analysis and the richness of linked open data to discover and recommend relevant, high-quality, free-licensed pictures for Wikidata items that don’t already have an image attached.
The number of images added to wiki projects since the beginning of 2015. Graph by Miriam Redi, data collected by Magnus Manske, CC0.
The number of Wikidata items is rapidly growing. There are now 2.5 million images in Wikidata, which outnumbers the number of images on English Wikipedia. In the past three years, the number of images contributed to Wikidata has grown at a much faster rate compared to its its sister projects.
While the volume of visual knowledge in Wikidata is now large relative to other projects, these 2.5 million images represent a tiny fraction of the material needed to visually represent all entries in a collaborative knowledge base. About 95 percent of Wikidata items currently lack an image statement. Although some types of entries—such as bibliographic items—don’t require an image, many do. In categories of items like ‘people,’only 17 percent of items have images. The same is true for ‘species’, where only 8 percent of entries have images. Many of these would benefit from a high-quality, relevant image.
Finding the right images is expensive!
Adding images to Wikidata items can be a tedious process. Editors adding visual contributions might have to search for the right picture among various repositories of free-licensed images. Our aim with this research project is to help make it easier for editors to find an appropriate image.
What we did
We designed an algorithm to automatically discover and recommend potentially relevant and high-quality images for pictureless Wikidata items. This consists of two simple steps:
- Relevant image discovery: First, given a Wikidata entry without an image, we search Wikipedia and its sister projects for potentially relevant image candidates. We retrieve all images in pages linked to the item. We also pull all images returning from querying Wikimedia Commons with the item label. We then exclude all images whose title does not match the item label (e.g., we would retain Mont Blanc and Dome du Gouter.jpg for the Mont Blanc entry) from the set of returned images. In the future, we are planning to design more complex algorithms to measure the relevance of an image to a Wikidata item, (i.e. the extent to which the image depicts the item.)
- Quality image ranking: To find the ‘best’ pictures among those discovered in the first step, we rank them according to their intrinsic photographic quality. To do so, we first need to score images in terms of photographic quality. We do this automatically, resorting to the most recent computer vision techniques. We train a classifier, i.e. a convolutional neural network (CNN) to distinguish between high and low quality images. More specifically, we provide the classifier examples of Quality Commons images and Random commons (lower quality) images. The CNN automatically learns from the image pixels how to classify quality images (More info about the model). In average, our model is able to correctly say if an image si high quality or not around 78 percent of the times.
Some examples of species items without images, together with our candidate images ranked by quality can be found on Meta-Wiki. While this project is currently in a pilot stage,we are planning to feed these image recommendations into existing tools for Wikidata visual enrichment, such as Fist and File Candidates.
Evaluation: good images are ranked in the top three
To get an idea of the effectiveness of our methodology for Wikidata visual enrichment, we performed an early evaluation based on historical data of Magnus’ Wikidata Distributed game. This platform allows editors to choose the best image for a Wikidata item given a set of candidate images. We retrieve Distributed Game data for around 66K Wikidata items of various categories. For each item, we get the set of candidate images proposed, as well as the picture manually selected by the user. We run our algorithm on these items: we discover relevant candidates and rank them by quality. We find that around 76% of the times, our algorithm would rank the manually chosen image is in the top three.
This tells us that, using this algorithm, that we may substantially reduce the search space for wikidata visual enrichment. Most of the times, we could filter out bad images and present editors with just 3 pictures to be inspected for visual enrichment of a Wikidata item.
Beyond Commons: Flickr
The aim of this research is to find the best possible pictorial representation of a Wikidata item. While Wikimedia Commons is the largest repository of free-licensed images in the world, and many Commons files are extremely valuable pieces of content, other image repositories such as Flickr or UNsplash also contain high quality free images. In a small-scale experiment based on image analysis, we discovered that only 0.1% of free Flickr images (of monuments) are already on Commons. In the future, we could leverage our technologies to discover and import high quality free-licensed images from Flickr.
Beyond Wikidata: Wikipedia
For the pilot stage of this project, we focused on Wikidata as the main collaborative repository for structured data. In the future, we would like to build on existing techniques to help with the visual enrichment of Wikidata’s sister projects such as Wikipedia. Learning from existing data, we could discover high-quality images that are relevant to Wikipedia articles or sections of articles, and recommend them to editors willing to use more images for knowledge sharing.
How to get involved
Inspect and play with some of the recommendations for Wikidata items of people by checking out our labs pages on this (1, 2). And read more about this work in our Meta-Wiki page.
Miriam Redi, Research Scientist
Wikimedia Foundation
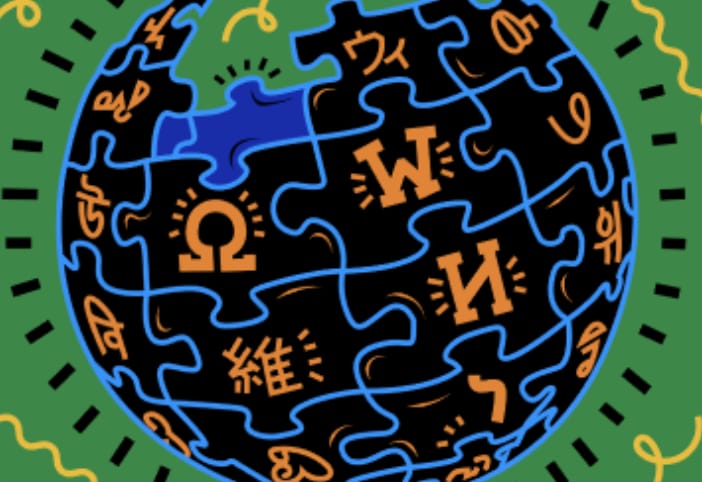
Can you help us translate this article?
In order for this article to reach as many people as possible we would like your help. Can you translate this article to get the message out?
Start translation