The Wikimedia Foundation Research shares an update to its 2019 Knowledge Gaps White Paper. With a continued commitment to knowledge equity, the update reflects on the past, present, and future of our research around Knowledge Gaps, provides a summary of our findings and contributions, and revises the roadmap for the next 3-5 years.
The update describes three main developments:
- Guiding Principles, a set of principles guiding our research in knowledge gaps: knowledge equity, multi-project focus, community-driven research, machine-in-the-loop, inclusivity, privacy, and openness.
- Consolidated Research Roadmap, consisting of three main directions: identify, measure, and bridge knowledge gaps.
- Ideas for Future Research: big research questions, spanning a 5 to 10-year horizon, which we would like to share with the research community.
This post summarizes the key points of each development. For more in-depth information about our revised roadmap, please refer to the full update (or its pdf version on Commons), and stay tuned for follow-up posts! Please share suggestions and feedback on the talk page. We are looking forward to hearing your thoughts on our knowledge gaps research!
Principles Guiding Knowledge Gaps Research
- Knowledge Equity. The Knowledge Equity principle is at the heart of the knowledge gaps research. Our aim is to provide tools and research to identify, measure, and address those knowledge gaps that prevent us from reaching knowledge equity.
- Beyond one project. We conduct research for Wikimedia projects including but not limited to Wikipedia, such as Wikidata, Wikimedia Commons, Wikisource, and Wiktionary.
- Community-driven research. We are inspired and influenced by Wikimedia communities. Our aim is to develop research and technologies that work in harmony with community principles and mechanisms.
- Inclusive research methods. Knowledge equity starts from the technologies we develop. To support a diverse community, our outputs are inclusive by design, and scalable across platforms, content types, languages, and cultures.
- Machine-in-the-loop. Our automated systems are designed to play a supporting role for editors, empowering them and improving their capacity. Editors and other users should keep full control of whether to adopt or reject algorithmic suggestions.
- Privacy. Our research is guided by the respect for privacy that is core to all Wikimedia projects.
- Openness. Freedom and Open Source is a guiding principle of our research. We follow the Wikimedia Foundation’s Open Access policy.
Our 3-5 years Research Roadmap: Identify, Measure, and Bridge Knowledge Gaps
Identify Knowledge Gaps
This direction focuses on developing systematic definitions of knowledge gaps and their context as the first step towards operationalizing knowledge equity. Our research is focusing on four main goals:
- Define Knowledge Gaps. We developed and are continuously improving the first taxonomy of Wikimedia knowledge gaps, a structured, systematic list of inequalities in Wikimedia projects across readers, contributors, and content.
- Define Barriers to Knowledge. We plan to create a taxonomy of barriers preventing people from accessing free knowledge.
- Understanding Readers and Contributors. We are working on understanding in-depth reader motivation, navigation patterns, and curiosity, as well as contributor workflows, to help uncover and prioritize new and existing knowledge gaps..
- The Role of Images and Multimedia. We are interested in studying the role of multimedia content in Wikimedia platforms, the extent of its presence, and its impact on navigation.
Measure Knowledge Gaps
Once knowledge gaps are systematically defined, the next challenge is to develop ways to measure them. Our goals for this direction are as follows:
- Quantify knowledge gaps. We defined high-level metrics, namely the tools, data, and logic needed to measure the knowledge gaps in the taxonomy, tested some readers and contributors metrics and we are currently working on metrics for the multimedia gap and the readability gap.
- Create snapshots of the state of gaps over time. We are currently deploying 5 demographics content gap metrics and are fostering the creation of new tools and research to systematically generate data about disparities in content, contributors, and readers.
- Build a tool to explore knowledge gaps data. We are building the knowledge gap index, an accessible and user-friendly interface, through which we hope to allow Wikimedians and researchers to monitor data about knowledge gaps, set targets and track the progress towards those goals.
Bridge Knowledge Gaps
This direction discusses ways to address Wikimedia knowledge gaps, informed by the systematic definitions and measurements described so far. We are working on two main research fronts:
- Discover, prioritize, and tag Wikimedia Content. Operating in harmony with community practices, we are developing and encouraging research around tools for content recommendation (for example list, building, link recommendation, image recommendation and section recommendation), prioritization (based on article importance) and tagging (see topic filters) that can help communities address gaps.
- Tools and systems to address knowledge gaps. Besides collaborating with WMF’s product teams to support the development of structured tasks, we are studying ways to promote equity and reduce biases through our recommender systems. We also developed a set of tools that facilitate the visualization and exploration of patterns in Wikimedia projects, and continue our collaborations with teams inside of WMF, the Wikimedia affiliates, and the developer community to build products that address knowledge gaps.
Beyond the 5-Year Horizon: Ideas for the Future
During our conversations, several ideas for research projects with a longer-term horizon emerged. We share them here with hope to generate interest and awareness around research questions that are crucial for the future of Wikimedia projects.
- Learning. One of the main motivations bringing people to Wikimedia sites is intrinsic learning. But are our content, tools, and recommender systems designed to foster and maximize learning?
- A Model of Wikipedia’s Complexity. Researchers have long studied ways to model complex systems such as climate change or social networks, and these models have been shown to be extremely useful in understanding the underlying mechanisms and providing quantitative forecasts. Can we have a model that reflects Wikipedia’s processes and can help answer questions about knowledge gaps and imagine extreme scenarios?
- Named Entity Recognition in images. Named Entity Recognition is a well-established NLP task that, given a piece of natural language text, such as a Wikipedia article, extracts semantic entities in a knowledge graph such as Wikidata. The recognition of named entities in text has been widely explored in the natural language processing field. But how can we classify highly granular semantics in images?
- New and External forms of knowledge While most of our work focuses on understanding gaps in Wikimedia spaces as of today, Wikipedia and its sister projects are in continuous evolution. Wikimedia projects do not live in isolation: they are an essential part of the larger web ecosystem. How does Wikipedia address the knowledge gaps of the broader web, and what forms of knowledge Wikimedia needs to acquire in order to fulfill its role in the web?
Acknowledgements
The Research team would like to thank everyone who has supported us throughout the years with the Knowledge Gaps research and who has given love and feedback to the Knowledge Gap projects: thank you all.
Funds for this research are provided by donors who give to the Wikimedia Foundation, by grants from the Siegel Family Endowment, and by a grant from the Argosy Foundation. Thank you!
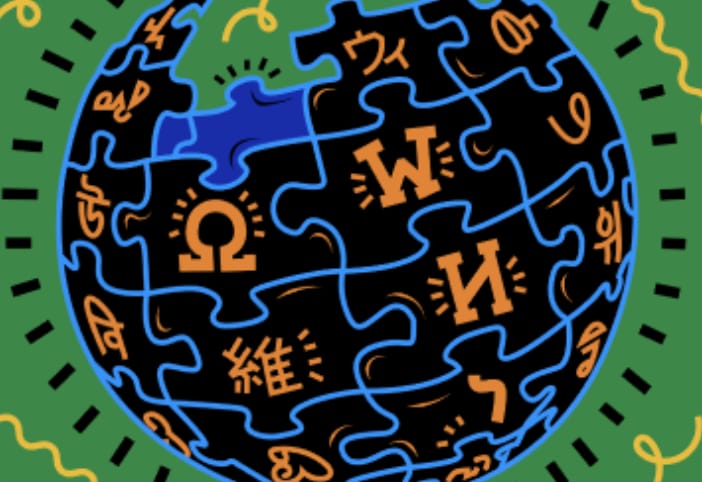
Can you help us translate this article?
In order for this article to reach as many people as possible we would like your help. Can you translate this article to get the message out?
Start translation