Contents
Medical articles on French Wikipedia have “high rate of veracity”
- Reviewed by Nicolas Jullien
A doctoral thesis[1] at Aix-Marseille University examined the accuracy of medical articles on the French Wikipedia. From the English abstract: “we selected a sample of 5 items (stroke, colon cancer, diabetes mellitus, vaccination and interruption of pregnancy) which we compare, assertion by assertion, with reference sources to confirm or refute each assertion. Results: Of the 5 articles, we analyzed 868 assertions. Of this total, 82.49% were verified by the referentials, 15.55% not verifiable due to lack of information and 1.96% contradicted by the referentials. Of the contradicted results, 10 corresponded to obsolete notions and 7 to errors, but mainly dealing with epidemiological or statistical data, thus not leading to a major risk when used, not recommended, on health. Conclusion: … This study of five medical articles finds a high rate of veracity with less than 2% incorrect information and more than 82% of information confirmed by scientific references. These results strongly argue that Wikipedia could be a reliable source of medical information, provided that it does not remain the only source used by people for that purpose.”
This medical PhD thesis is a very well documented analysis of the questions raised by the publication of medical information on Wikipedia. Although the findings, summarized in the abstract, will not be new to those who know Wikipedia well, it presents a good review of the literature on the topic of medical accuracy, and also of the purpose of Wikipedia (not a professional encyclopedia, but a form of popular science, an introduction, and some links to go further). This document is in French.
Assessing article quality and popularity across 44 Wikipedia language versions
- Reviewed by Nicolas Jullien
This is the topic of a paper in the journal Informatics[2]. From the English abstract: “Our research has showed that in language sensitive topics, the quality of information can be relatively better in the relevant language versions. However, in most cases, it is difficult for the Wikipedia readers to determine the language affiliation of the described subject. Additionally, each language edition of Wikipedia can have own rules in the manual assessing of the content’s quality. There are also differences in grading schemes between language versions: some use a 6–8 grade system to assess articles, and some are limited to 2–3. This makes automatic quality comparison of articles between various languages a challenging task, particularly if we take into account a large number of unassessed articles; some of the Wikipedia language editions have over 99% of articles without a quality grade. The paper presents the results of a relative quality and popularity assessment of over 28 million articles in 44 selected language versions. Comparative analysis of the quality and the popularity of articles in popular topics was also conducted. Additionally, the correlation between quality and popularity of Wikipedia articles of selected topics in various languages was investigated. The proposed method allows us to find articles with information of better quality that can be used to automatically enrich other language editions of Wikipedia.”
Regarding the quality metrics, I salute the coverage in terms of languages, which allows to go beyond the “official” automated evaluation provided by the Wikimedia Foundation (ORES) that is only available on some big language projects. As the authors explained, this part is mostly based on a work already published, but fairly extended. It also proposes some solutions to the quality comparisons between different languages, and takes into account the variations of perspectives between different cultures.
It also opens a discussion about the popularity of articles, and how this can help to choose which master language has to be chosen when an article exists. Although this part is just at its beginning, their discussion makes the next step for their work, looking forward.
- Reviewed by FULBERT
This theoretical paper[3] explored ambiguous relationships between credibility, trust, and authority in library and information sciences and how they are related to perceived accuracy in information sources. Credibility is linked to trust, necessary when we seek to learn from or convey information between people. This is complicated when the authority of a source is considered, as personal or institutional levels of expertise increase the ability to speak with greater credibility.
The literature about how this works with knowledge and information on the Web is inconsistent, and as a result this work sought to develop a unified approach through a new model. As credibility, trust, and authority are distinct concepts that are frequently used together inconsistently, they were explored through how Wikipedia is used and perceived. While Wikipedia is considered highly accurate, trust in it is average while its credibility is at times suspect.
Sahut and Tricot developed the authority, trust and credibility (ATC) model, where “knowledge institutions confer authority to a source, this authority ensures trust, which ensures the credibility of the information.” As a result, “the credibility of the information builds trust, which builds the authority of the source.” This model can be useful when applying to the citation of sources in Wikipedia, as it helps explain how the practice of providing citations in Wikipedia increases credibility and thus encourages trust, “linking content to existing knowledge sources and institutions.”
The ATC model is a helpful framework for explaining how Wikipedia, with its enormous readership, continues to suffer from challenges to being perceived as an authority due to its inconsistencies in article citations and references. This theorizes that filling these gaps will increase authority and thus the reputation of Wikipedia itself.
Conferences and events
Academia and Wikipedia: Critical Perspectives in Education and Research
A call for papers has been published for a conference titled “Academia and Wikipedia: Critical Perspectives in Education and Research”, to be held on June 18, 2018, at Maynooth University in the Republic of Ireland. The organizers describe it as “a one-day conference that aims to investigate how researchers and educators use and interrogate Wikipedia. The conference is an opportunity to present research into and from Wikipedia; research about Wikipedia, or research that uses Wikipedia as a data object”.
Wiki Workshop 2018
The fifth edition of Wiki Workshop will take place in Lyon, France on April 24, 2018, as part of The Web Conference 2018. Wiki Workshop brings together researchers exploring all aspects of Wikimedia websites, such as Wikipedia, Wikidata, and Wikimedia Commons. The call for papers is now available. The submission deadline for papers to appear in the proceedings of the conference is January 28, all other papers on March 11.
See the research events page on Meta-wiki for other upcoming conferences and events, including submission deadlines.
Other recent publications
Other recent publications that could not be covered in time for this issue include the items listed below. contributions are always welcome for reviewing or summarizing newly published research.
- Compiled by Tilman Bayer
OpenSym 2017
- “What do Wikidata and Wikipedia have in common?: An analysis of their use of external references”[4] From the abstract: “Our findings show that while only a small number of sources is directly reused across Wikidata and Wikipedia, references often point to the same domain. Furthermore, Wikidata appears to use less Anglo-American-centred sources.”
- “A glimpse into Babel: An analysis of multilinguality in Wikidata”[5] From the abstract: “we explore the state of languages in Wikidata as of now, especially in regard to its ontology, and the relationship to Wikipedia. Furthermore, we set the multilinguality of Wikidata in the context of the real world by comparing it to the distribution of native speakers. We find an existing language maldistribution, which is less urgent in the ontology, and promising results for future improvements.”
- “Before the sense of ‘we’: Identity work as a bridge from mass collaboration to group emergence”[6] From the paper: “… From these interviews, we identified that a Featured Article (FA) collaboration that had occurred in 2007 in the “Whooper Swan” Wikipedia article, was very important for the actions of later group work. The focus of this paper is around this foundational article.”
- “Interpolating quality dynamics in Wikipedia and demonstrating the Keilana effect”[7] From the abstract: “I describe a method for measuring article quality in Wikipedia historically and at a finer granularity than was previously possible. I use this method to demonstrate an important coverage dynamic in Wikipedia (specifically, articles about women scientists) and offer this method, dataset, and open API to the research community studying Wikipedia quality dynamics.” (see also research project page on Meta-wiki)
See also our earlier coverage of another OpenSym 2017 paper: “Improved article quality predictions with deep learning“
OpenSym 2016
- “Mining team characteristics to predict Wikipedia article quality”[8] From the abstract: “The experiment involved obtaining the Spanish Wikipedia database dump and applying different data mining techniques suitable for large data sets to label the whole set of articles according to their quality (comparing them with the Featured/Good Articles, or FA/GA). Then we created the attributes that describe the characteristics of the team who produced the articles and using decision tree methods, we obtained the most relevant characteristics of the teams that produced FA/GA. The team’s maximum efficiency and the total length of contribution are the most important predictors.”
- “Predicting the quality of user contributions via LSTMs”[9] From the discussion section: “We have presented a machine-learning approach for predicting the quality of Wikipedia revisions that can leverage the complete contribution history of users when making predictions about the quality of their latest contribution. Rather than using ad-hoc summary features computed on the basis of user’s contribution history, our approach can take as input directly the information on all the edits performed by the user [e.g. features such as “Time interval to previous revision on page”, the number of characters added or removed, “Spread of change within the page”, “upper case/ lower case ratio”, and “day of week”]. Our approach leverages the power of LSTMs (long-short term memory neural nets) for processing the variable-length contribution history of users.”
- “Monitoring the gender gap with Wikidata human gender indicators”[10] From the abstract: “The gender gap in Wikipedia’s content, specifically in the representation of women in biographies, is well-known but has been difficult to measure. Furthermore the impacts of efforts to address this gender gap have received little attention. To investigate we use Wikidata, the database that feeds Wikipedia, and introduce the “Wikidata Human Gender Indicators” (WHGI), a free and open-source, longitudinal, biographical dataset monitoring gender disparities across time, space, culture, occupation and language. Through these lenses we show how the representation of women is changing along 11 dimensions. Validations of WHGI are presented against three exogenous datasets: the world’s historical population, “traditional” gender-disparity indices (GDI, GEI, GGGI and SIGI), and occupational gender according to the US Bureau of Labor Statistics.” (see also Wikimedia Foundation grant page)
- “An empirical evaluation of property recommender systems for Wikidata and collaborative knowledge bases”[11] From the abstract: “Users who actively enter, review and revise data on Wikidata are assisted by a property suggesting system which provides users with properties that might also be applicable to a given item. … We compare the [recommendation] approach currently facilitated on Wikidata with two state-of-the-art recommendation approaches stemming from the field of RDF recommender systems and collaborative information systems. Further, we also evaluate hybrid recommender systems combining these approaches. Our evaluations show that the current recommendation algorithm works well in regards to recall and precision, reaching a recall of 79.71% and a precision of 27.97%.”
- “Medical science in Wikipedia: The construction of scientific knowledge in open science projects”[12] From the abstract: “The goal of my research is to build a theoretical framework to explain the dynamic of knowledge building in crowd-sourcing based environments like Wikipedia and judge the trustworthiness of the medical articles based on the dynamic network data. By applying actor–network theory and social network analysis, the contribution of my research is theoretical and practical as to build a theory on the dynamics of knowledge building in Wikipedia across times and to offer insights for developing citizen science crowd-sourcing platforms by better understanding how editors interact to build health science content.”
- ‘“Comparing OSM area-boundary data to DBpedia”[13] From the abstract: “OpenStreetMap (OSM) is a well known and widely used data source for geographic data. This kind of data can also be found in Wikipedia in the form of geographic locations, such as cities or countries. Next to the geographic coordinates, also statistical data about the area of these elements can be present. … in this paper OSM data of different countries are used to calculate the area of valid boundary (multi-) polygons and are then compared to the respective DBpedia (a large-scale knowledge base extract from Wikipedia) entries.”
See also our earlier coverage of another OpenSym 2016 paper: “Making it easier to navigate within article networks via better wikilinks“
Diverse other papers, relating to structured data
- “Scholia and scientometrics with Wikidata”[14] From the abstract: “Scholia is a tool to handle scientific bibliographic information in Wikidata. The Scholia Web service creates on-the-fly scholarly profiles for researchers, organizations, journals, publishers, individual scholarly works, and for research topics. To collect the data, it queries the SPARQL-based Wikidata Query Service.”
- “Linking Wikidata to the rest of the Semantic Web”[15]
- “Chaudron: Extending DBpedia with measurement”[16] From the abstract: “We propose an alternative extraction to the traditional mapping creation from Wikipedia dump, by also using the rendered HTML to avoid the template transclusion issue. This dataset extends DBpedia with more than 3.9 million triples and 949.000 measurements on every domain covered by DBpedia. […] An extensive evaluation against DBpedia and Wikidata shows that our approach largely surpasses its competitors for measurement extraction on Wikipedia Infoboxes. Chaudron exhibits a F1-score of .89 while DBpedia and Wikidata respectively reach 0.38 and 0.10 on this extraction task.”
- “Assessing and Improving Domain Knowledge Representation in DBpedia”[17] From the abstract: “… we assess the quality of DBpedia for domain knowledge representation. Our results show that DBpedia has still much room for improvement in this regard, especially for the description of concepts and their linkage with the DBpedia ontology. Based on this analysis, we leverage open relation extraction and the information already available on DBpedia to partly correct the issue, by providing novel relations extracted from Wikipedia abstracts and discovering entity types using the dbo:type predicate …”
- “A Case Study of Summarizing and Normalizing the Properties of DBpedia Building Instances”[18] From the abstract: “The DBpedia ontology [holds] information for thousands of important buildings and monuments, thus making DBpedia an international digital repository of the architectural heritage. This knowledge for these architectural structures, in order to be fully exploited for academic research and other purposes, must be homogenized, as its richest source – Wikipedia infobox template system – is a heterogeneous and non-standardized environment. The work presented below summarizes the most widely used properties for buildings, categorizes and highlights structural and semantic heterogeneities allowing DBpedia’s users a full exploitation of the available information.”
- “Experience: Type alignment on DBpedia and Freebase”[19] From the abstract: “… instances of many different types (e.g. Person) can be found in published [ linked open data ] datasets. Type alignment is the problem of automatically matching types (in a possibly many-many fashion) between two such datasets. Type alignment is an important preprocessing step in instance matching. Instance matching concerns identifying pairs of instances referring to the same underlying entity. By performing type alignment a priori, only instances conforming to aligned types are processed together, leading to significant savings. This article describes a type alignment experience with two large-scale cross-domain RDF knowledge graphs, DBpedia and Freebase, that contain hundreds, or even thousands, of unique types. Specifically, we present a MapReduce-based type alignment algorithm … “
- “High-Throughput and Language-Agnostic Entity Disambiguation and Linking on User Generated Data”[20] From the preprint (which contains no mention of Wikidata): “Our KB [ knowledge base] consists of about 1 million Freebase machine ids for entities. These were chosen from a subset of all Freebase entities that map to Wikipedia entities. We prefer to use Freebase rather than Wikipedia as our KB since in Freebase, the same id represents a unique entity across multiple languages… …we generated a ground truth data set for our EDL system, the Densely Annotated Wikipedia Text (DAWT), using densely Wikified or annotated Wikipedia articles. Wikification is entity linking with Wikipedia as the KB. We started with Wikipedia data dumps, which were further enriched by introducing more hyperlinks in the existing document structure. […] As a last step, the hyperlinks to Wikipedia articles in a specific language were replaced with links to their Freebase ids to adapt to our KB. … We also plan to migrate to Wikipedia as our KB.”
- “Managing and Consuming Completeness Information for Wikidata Using COOL-WD”[21] From the abstract: “… we discuss how to manage and consume meta-information about completeness for Wikidata. […] We demonstrate the applicability of our approach via COOL-WD (http://cool-wd.inf.unibz.it/), a completeness tool for Wikidata, which at the moment collects around 10,000 real completeness statements.” (see also related paper)
- “Querying Wikidata: Comparing SPARQL, Relational and Graph Databases”[22] From the abstract: “… we experimentally compare the efficiency of various database engines for the purposes of querying the Wikidata knowledge-base…”
- “Reifying RDF: What Works Well With Wikidata?”[23] From the abstract: “… we compare various options for reifying RDF triples. We are motivated by the goal of representing Wikidata as RDF, which would allow legacy Semantic Web languages, techniques and tools – for example, SPARQL engines – to be used for Wikidata. However, Wikidata annotates statements with qualifiers and references, which require some notion of reification to model in RDF. We thus investigate four such options: …” (A SPARQL-based search engine for Wikidata has since become available.)
References
- ↑ Antonini, Sébastien (2017-06-22). “Étude de la véracité des articles médicaux sur Wikipédia”. Aix Marseille Université.
- ↑ Lewoniewski, Włodzimierz; Krzysztof, Węcel; Abramowicz, Witold (2017-06-22). “Relative Quality and Popularity Evaluation of Multilingual Wikipedia”. Informatics 2017, 4(4), 43.
- ↑ Sahut, Gilles; Tricot, André (2017-10-31). “Wikipedia: An opportunity to rethink the links between sources’ credibility, trust, and authority”. First Monday 22 (11). ISSN 1396-0466. doi:10.5210/fm.v22i11.7108. Retrieved 2017-12-17.
- ↑ Piscopo, Alessandro; Vougiouklis, Pavlos; Kaffee, Lucie-Aimée; Phethean, Christopher; Hare, Jonathon; Simperl, Elena (2017). What do Wikidata and Wikipedia have in common?: An analysis of their use of external references (PDF). OpenSym ’17. New York, NY, USA: ACM. pp. 1–1–1:10. ISBN 9781450351874. doi:10.1145/3125433.3125445.
- ↑ Kaffee, Lucie-Aimée; Piscopo, Alessandro; Vougiouklis, Pavlos; Simperl, Elena; Carr, Leslie; Pintscher, Lydia (2017). A glimpse into Babel: An analysis of multilinguality in Wikidata (PDF). OpenSym ’17. New York, NY, USA: ACM. pp. 14–1–14:5. ISBN 9781450351874. doi:10.1145/3125433.3125465.
- ↑ Lanamäki, Arto; Lindman, Juho (2017). Before the sense of ‘we’: Identity work as a bridge from mass collaboration to group emergence (PDF). OpenSym ’17. New York, NY, USA: ACM. pp. 5–1–5:9. ISBN 9781450351874. doi:10.1145/3125433.3125451.
- ↑ Halfaker, Aaron (2017). Interpolating quality dynamics in Wikipedia and demonstrating the Keilana effect (PDF). OpenSym ’17. New York, NY, USA: ACM. pp. 19–1–19:9. ISBN 9781450351874. doi:10.1145/3125433.3125475.
- ↑ Betancourt, Grace Gimon; Segnine, Armando; Trabuco, Carlos; Rezgui, Amira; Jullien, Nicolas (2016). Mining team characteristics to predict Wikipedia article quality. OpenSym ’16. New York, NY, USA: ACM. pp. 15–1–15:9. ISBN 9781450344517. doi:10.1145/2957792.2971802.
- ↑ Agrawal, Rakshit; deAlfaro, Luca (2016). Predicting the quality of user contributions via LSTMs (PDF). OpenSym ’16. New York, NY, USA: ACM. pp. 19–1–19:10. ISBN 9781450344517. doi:10.1145/2957792.2957811.
- ↑ Klein, Maximilian; Konieczny, Piotr; Zhu, Haiyi; Rai, Vivek; Gupta, Harsh (2016). Monitoring the gender gap with Wikidata human gender indicators (PDF). OpenSym 2016. Berlin, Germany. p. 9.
- ↑ Zangerle, Eva; Gassler, Wolfgang; Pichl, Martin; Steinhauser, Stefan; Specht, Günther (2016). An empirical evaluation of property recommender systems for Wikidata and collaborative knowledge bases (PDF). OpenSym ’16. New York, NY, USA: ACM. pp. 18–1–18:8. ISBN 9781450344517. doi:10.1145/2957792.2957804.
- ↑ Tamime, Reham Al; Hall, Wendy; Giordano, Richard (2016). Medical science in Wikipedia: The construction of scientific knowledge in open science projects (PDF). OpenSym ’16. New York, NY, USA: ACM. pp. 4–1–4:4. ISBN 9781450344814. doi:10.1145/2962132.2962141. (extended abstract)
- ↑ Silbernagl, Doris; Krismer, Nikolaus; Specht, Günther (2016). Comparing OSM area-boundary data to DBpedia (PDF). OpenSym ’16. New York, NY, USA: ACM. pp. 11–1–11:4. ISBN 9781450344517. doi:10.1145/2957792.2957806.
- ↑ Nielsen, Finn Årup; Mietchen, Daniel; Willighagen, Egon (2017-05-28). Scholia, Scientometrics and Wikidata. European Semantic Web Conference. Lecture Notes in Computer Science. Springer, Cham. pp. 237–259. ISBN 9783319704067. doi:10.1007/978-3-319-70407-4_36.
- ↑ Andra Waagmeester, Egon Willighagen, Núria Queralt Rosinach, Elvira Mitraka, Sebastian Burgstaller-Muehlbacher, Tim E. Putman, Julia Turner, Lynn M Schriml, Paul Pavlidis, Andrew I Su, and Benjamin M Good: Linking Wikidata to the rest of the Semantic Web. Proceedings of the 9th International Conference Semantic Web Applications and Tools for Life Sciences. Amsterdam, The Netherlands, December 5-8, 2016. (conference poster)
- ↑ Subercaze, Julien (May 2017). Chaudron: Extending DBpedia with measurement. Portoroz, Slovenia: Eva Blomqvist, Diana Maynard, Aldo Gangemi.
- ↑ Ludovic Font A, Amal Zouaq A, B, Michel Gagnon: Assessing and Improving Domain Knowledge Representation in DBpedia
- ↑ Agathos, Michail; Kalogeros, Eleftherios; Kapidakis, Sarantos (2016-09-05). “A Case Study of Summarizing and Normalizing the Properties of DBpedia Building Instances”. In Norbert Fuhr, László Kovács, Thomas Risse, Wolfgang Nejdl (eds.). Research and Advanced Technology for Digital Libraries. Lecture Notes in Computer Science. Springer International Publishing. pp. 398–404. ISBN 9783319439969. Retrieved 2016-08-27.
- ↑ KEJRIWAL, MAYANK; MIRANKER, DANIEL P. (2016). “Experience: Type alignment on DBpedia and Freebase” (PDF). ACM: 10.
- ↑ Bhargava, Preeti; Spasojevic, Nemanja; Hu, Guoning (2017-03-13). “High-Throughput and Language-Agnostic Entity Disambiguation and Linking on User Generated Data”. arXiv:1703.04498 [cs].
- ↑ Prasojo, Radityo Eko; Darari, Fariz; Razniewski, Simon; Nutt, Werner. Managing and Consuming Completeness Information for Wikidata Using COOL-WD (PDF). KRDB, Free University of Bozen-Bolzano, 39100, Italy.
- ↑ Hernández, Daniel; Hogan, Aidan; Riveros, Cristian; Rojas, Carlos; Zerega, Enzo (2016-10-17). Querying Wikidata: Comparing SPARQL, Relational and Graph Databases. International Semantic Web Conference. Lecture Notes in Computer Science. Springer, Cham. pp. 88–103. ISBN 9783319465463. doi:10.1007/978-3-319-46547-0_10.
author’s preprint
- ↑ Hernández, Daniel; Hogan, Aidan; Krötzsch, Markus (2015). “Reifying RDF: What Works Well With Wikidata?”. Reifying RDF: What Works Well With Wikidata?. Proceedings of the 11th International Workshop on Scalable Semantic Web Knowledge Base Systems,. 1457 of CEUR Workshop Proceedings. pp. 32–47.
Wikimedia Research Newsletter
Vol: 7 • Issue: 9 • September 2017
This newsletter is brought to you by the Wikimedia Research Committee and The Signpost
Subscribe: Email
• [archives] [signpost edition] [contribute] [research index]
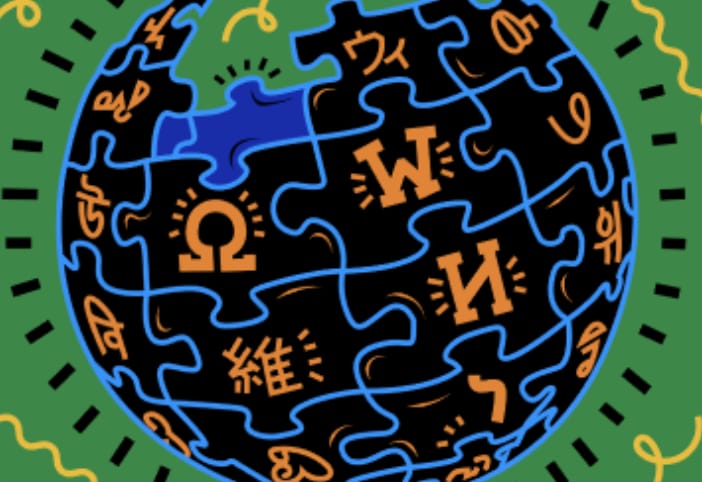
Can you help us translate this article?
In order for this article to reach as many people as possible we would like your help. Can you translate this article to get the message out?
Start translation