
For more than 10 years now, cultural institutions around the world have partnered with Wikimedians to make their collections more visible and to encourage re-use via Wikimedia platforms. Collaborations of this kind, GLAM-Wiki projects (with Galleries, Libraries, Archives and Museums), often use Wikipedia and Wikimedia Commons as platforms. Images of cultural collections are uploaded to Wikimedia’s media repository Wikimedia Commons and are re-used as illustrations in Wikipedia articles.
For several years, a growing number of GLAM-Wiki partnerships also work with Wikidata, the free, multilingual knowledge base of the Wikimedia ecosystem. Cultural institutions and Wikimedians upload data about cultural collections to Wikidata: it provides an accessible way to publish collections data as Linked Open Data, and makes the collection data multilingual, re-usable and discoverable across the web. Since 2019, files on Wikimedia Commons can now also be described with multilingual structured data from Wikidata. This will make the (structured) data component of GLAM-Wiki collaborations even more prominent in the future.
Photo of the buckle ‘Plume de Paon’ by Philippe Wolfers, ca. 1898. Photo Royal Museums of Art and History (Brussels), CC BY-SA 4.0 Structured data for this image.
“If roundtrips work, that will sell everyone on it—no questions asked!”
With this shift to more (Linked Open) data activities in GLAM-Wiki, we see new requests from the GLAM sector. Cultural institutions—especially those who are able to export and import data from and into their collections databases, and who maintain their own APIs—are increasingly interested in ‘metadata roundtripping’: synchronization between the institution’s collection database and the data about their collections that lives on Wikimedia sites.
This data roundtripping can happen in two directions:
- Wikimedia → institution. When an institution contributes data and media files to Wikidata and Wikimedia Commons, Wikimedians can enrich this content: they can add translations, transcribe texts, add descriptive metadata to the data entities and files (for instance: what is depicted in a photo or painting?) or even correct information (for instance, volunteers on Wikidata have helped institutions find duplicate records in their databases, or have helped identify the correct creators of works). For many cultural institutions, it is interesting to verify these modifications and to ‘feed’ correct and valuable improvements back into their own collections database.
- Institution → Wikimedia. Institutional collections grow, and change, all the time. Museums, libraries and archives acquire new collection items. Sometimes, information about existing collections is updated (for instance in the case of re-attributions, when research shows that a work was actually created by someone else than originally thought). Institutions who work very frequently with Wikimedia projects are interested in created automatic update systems, where modifications and new items in their own databases will be automatically uploaded to Wikimedia sites.
Recently, Jan Dittrich, UX Designer at Wikimedia Deutschland, has interviewed staff members of different GLAM institutions, asking them how they engage with Wikidata, their motivations, activities and problems. Out of 16 respondents, 7 indicated interest in data roundtripping. Several stated that they are motivated by the potential of crowdsourced improvements to their data by Wikimedians; three respondents mentioned that their own data may have quality problems. One interviewee even said: “If roundtrips work, that will sell everyone on it – no questions asked!”
A Swedish pilot project: improving metadata and feeding it back to institutions
The Swedish National Heritage Board (Riksantikvarieämbetet / RAÄ) supports Swedish institutions in their digital activities, and engages with Wikimedia platforms in that process. In the first half of 2019, RAÄ has investigated the popular request around metadata roundtripping, with a focus on the ‘Wikimedia → institution’ type, and on media files on Wikimedia Commons, in the project Wikimedia Commons Data Roundtripping. What kind of additional metadata can, and do, Wikimedians add to these files, and how can structured data provide added value? Are these enrichments good and interesting enough? What types of enrichments are institutions themselves interested in? And how easy or difficult would it be to feed these improvements back to the source institution?
In order to investigate and test these questions, RAÄ first commissioned a research report by Maarten Zeinstra (IP Squared), who held a survey and interviews with GLAM staff. Insights and recommendations from the research report include:
- GLAMs are interested in altered metadata of their files, in translations, and in new contextual metadata of the files (such as depicted people, places and other things).
- In order to help GLAMs trust the added metadata more, it would be good to present some information about the users who added the new metadata: for instance, are they experienced users who rarely get reverted?
- In order to make the re-ingestion of improvements technically easy, it’s best to present an export of the modified data in a very simple format—such as a .csv file—that can be processed by many types of databases.
- It would be valuable to design and present structured data Wikimedia Commons (and Wikidata) as authority files themselves; this will lower the barrier for adoption by cultural institutions.
In a second phase, RAÄ performed three pilot projects to test the potential of data roundtripping in practice.
A first pilot focused on translations, with glass plate photographs of Swedish actors, from the collections of the Swedish Performing Arts Agency.
Using a lightweight tool, users were invited to translate Swedish descriptions of the photographs to English, aided by Google Translate. One interesting observation from this pilot: automated translations tend to make users pay less attention to the quality of the texts. In some cases, users would accept faulty translations and other errors.
The second pilot project focused on quite specialized enrichments: authority data (links to other databases) added by Wikidata volunteers to Wikidata entities relevant for the Swedish Nationalmuseum were imported back to the Nationalmuseum’s database. No errors were found when checking various random items, and this import had the potential to introduce improvements in both the museum’s database and on Wikidata, by exposing confusions between various artist names, and discovering duplicates.
The third and last pilot project made use of (the then brand new) Structured Data on Wikimedia Commons functionalities: users were invited to add selected Depicts (P180) statements to a selection of fashion-related images from the Nordic Museum. The set of potential Depicts ‘tags’ was kept restricted to a small subset of Wikidata items: only terms from the Europeana Fashion vocabulary, which is also used by the museum itself.
After the tagging exercise, users indicated that they wanted to use other, specific terms from Wikidata outside the Europeana Fashion vocabulary. While using such a vocabulary will make it easier for an institution to ingest the improvements back to its own database, the limitation also prevents potentially very valuable new insights about the collection. According to the museum, the quality of contributions varied significantly per user—some users were very knowledgeable in costume history and added valuable and specific terms, while others only added very generic tags (‘hat’ vs ‘top hat’).
Conclusions
The final research report mentions various conclusions and recommendations, of which I’m highlighting several that are directly relevant to data roundtripping.
Importance of provenance
Storing and showing data provenance is important, especially in situations where crowdsourcing and machine learning, AI and machine translation are involved. In order to help assessment of data quality, it is important to explicitly indicate that a certain edit was informed by an algorithm; but it is also very valuable to be able to trace specific edits back to specific (trusted or less trusted) users.
Ease of export and import
On Wikidata’s side, the Wikidata Query Service offers an easy, extremely flexible method to retrieve improved data, allowing to select the data and export format that is useful for an institution. In the course of this pilot project by RAÄ, museum staff introduced to the Wikidata Query Service were able to work with it after only a short introduction. As soon as Wikimedia Commons will be equipped with its own operational query engine as well, retrieving structured data improvements from Commons will become similarly easy.
But on the institutions’ side, many different types of collections database software are used. Not all are able to easily import improved metadata. Some museums need to ask for help from vendors or other third parties to be able to import data in their systems. There is certainly a need for advocacy towards institutions and vendors to allow for easier data imports on the institutions’ side.
The potential of microcontributions and targeted tools
The research report also recommends to design and experiment with specific, targeted workflows for crowdsourcing and microcontributions. In fact, recently two such new enrichment tools for contextual metadata were developed in the Wikimedia community that deserve special attention, and probably also some future research and follow-up.
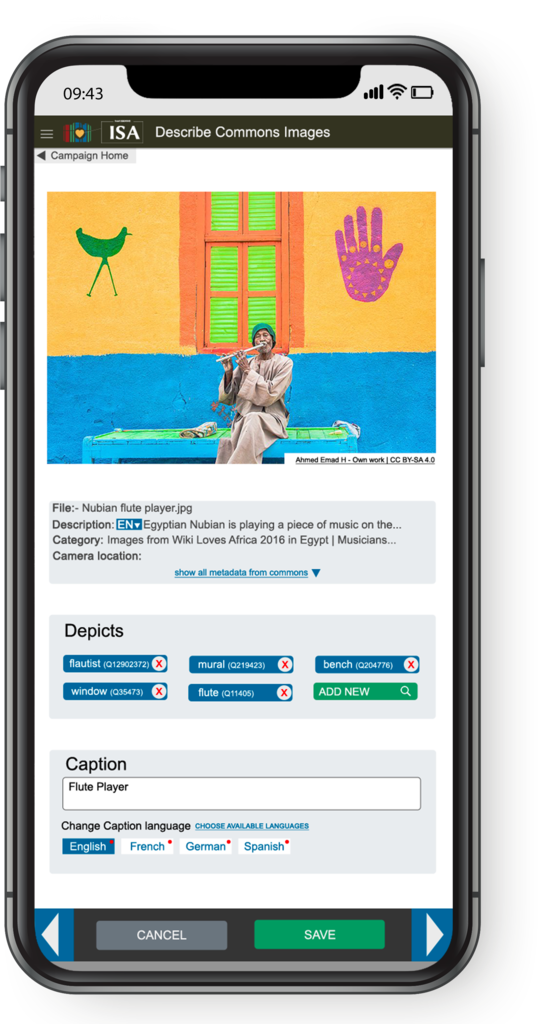
It will be interesting to investigate whether enrichments by these tools will prove to be interesting enough for cultural institutions to re-ingest them in their own databases—and, if that is not the case, how the design of such tools can be modified so that the contributions become of higher quality, more relevant, and more interesting.
The Metropolitan Museum of Art’s pioneering data roundtripping workflow
New York’s Metropolitan Museum of Art (“The Met”) has engaged with Wikimedia projects for a long time already. Richard Knipel (User:Pharos), The Met’s Wikipedian in Residence, has uploaded images for large parts of The Met’s collection to Wikimedia Commons, and has created the corresponding Wikidata items for the works. He also regularly organizes thematic edit-a-thons and challenges, in order to encourage more and better content about The Met’s collections on Wikimedia projects.
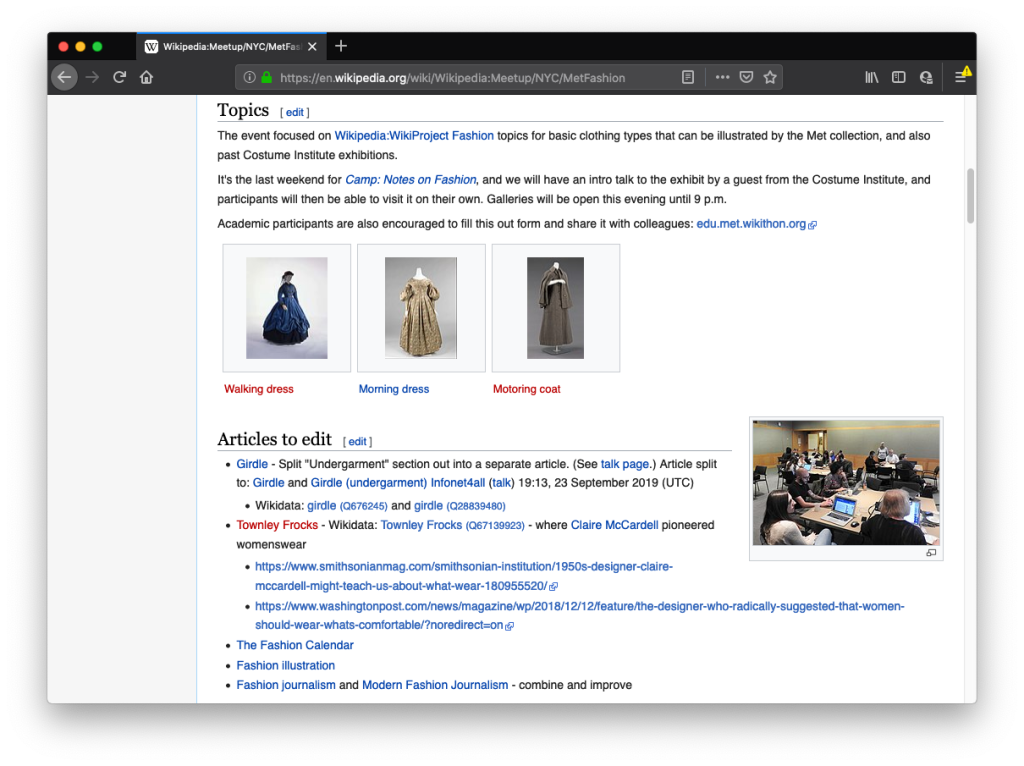
The Met has systemically engaged with Wikimedia for many years, and does so for strategic reasons. On several occasions, the museum—like many similar larger institutions and cultural aggregators around the world—has clearly expressed the wish to make the connection between its own collections databases and the Wikimedia ecosystem more automated.
“We also need to look at more integrated and sustainable models for exchanging data between cultural institutions and the Wikimedia platforms, and we believe that Wikidata may provide this opportunity. Wikidata is becoming a nearly universal platform for connecting data shared by institutions about their collections. It makes practical something the cultural heritage sector has been discussing and experimenting with for years—the idea of an interconnected web of “linked open data” that bridges the concepts, vocabularies, and languages that institutions use to describe their holdings. We see this work with Wikidata as the next threshold for our collaboration: a way to unite The Met collection with related artworks from museums and institutions across the world. A critical part of that work will be establishing an automated and scalable means by which data from cultural institutions can be seamlessly ingested by Wikidata, and synchronized to the Commons on an ongoing basis, removing the need for time-intensive, manual data upload and update processes.”
—Loic Tallon and Katherine Maher, Wikimedia and The Met: A Shared Digital Vision
In the past months, Andrew Lih—The Met’s Wikimedia Strategist—has developed a technical architecture for this.
- On the museum’s side, data from The Met’s API and csv is exported and converted to a Python (pandas) dataframe;
- On the Wikidata side, a SPARQL data export of The Met’s objects from Wikidata in json format is converted to a Python dataframe as well;
- Next, in a third, bi-directional comparison (diff), both dataframes are compared;
- Necessary updates to Wikidata are then exported to, and performed by, the QuickStatements batch editing tool, or with a bot (Pywikibot).
- Mappings between The Met’s metadata and Wikidata (for instance vocabulary terms and names of creators) take place in a Google spreadsheet, which is updated and maintained by curators from The Met and by knowledgeable Wikidata volunteers.
As The Met continues to extensively describe its images on Wikimedia Commons with structured data, it will be an interesting exercise to enhance this synchronization mechanism to make sure that this data on Commons will be kept in sync as well.
As mentioned above, many institutions have expressed interest in such automated workflows. While The Met’s general architecture and workflow is partially replicable on a higher level by many institutions, a ‘plug and play’ solution that immediately and automatically works for any institution is nontrivial. Each cultural institution maintains its own specialized data model and way of encoding person names, dates, keywords… (for examples, see this Python code (PAWS) notebook by Andrew Lih). Even if institutions strictly adhere to sector-wide metadata standards, it is likely that custom adjustments will always be needed to make the synchronization work. It will be interesting to work towards a generic technical architecture that works for many!
“GLAM-Wiki roundtripping is extremely powerful, especially for museums that are undergoing the enormous task of normalizing and integrating their linked open data sets. We are seeing GLAM institutions engage with structured Wikimedia content in a way that was not possible even six or seven years ago. It is realizing the promise of the semantic web as originally envisioned by Tim Berners-Lee as a rich dialogue of databases reinforcing and improving each other.”
—Andrew Lih, quote based on his Ignite MCN talk in November 2019.
This blog post was written with significant input by Jan Dittrich, Susanna Ånäs, Albin Larsson, and Andrew Lih. Thank you! ❤️ It is part of a short series of blog posts on Wikimedia Space about GLAM pilot projects with Structured Data on Wikimedia Commons. Earlier posts are:
- Introducing ISA – A cool tool for adding structured data on Commons by Isla Haddow-Flood
- How we helped a small art museum to increase the impact of its collections, with Wikimedia projects and structured data by Sandra Fauconnier
- How can Structured Data on Commons, Wikidata, and Wikisource walk hand in hand? A pilot project with Punjabi Qisse by Satdeep Gill
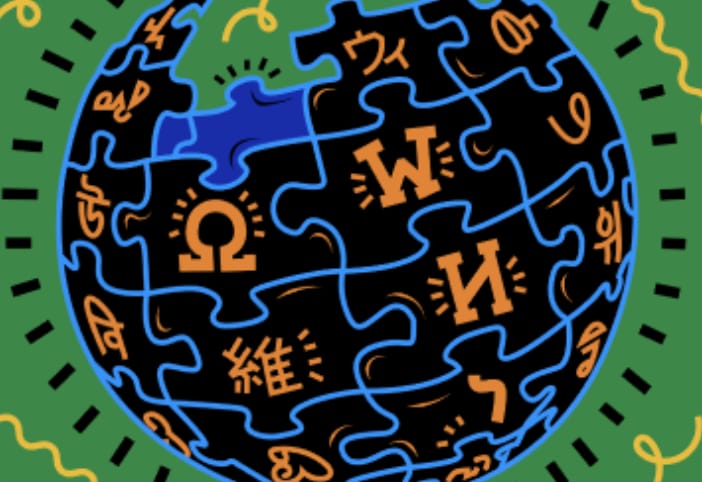
Can you help us translate this article?
In order for this article to reach as many people as possible we would like your help. Can you translate this article to get the message out?
Start translation